The Curious Case of Generative AI: A Paradigm Shift?
(This is an opinion piece)
As we look at the broader landscape of artificial intelligence, it's clear that the advent of generative AI is driving significant changes across multiple sectors. Generative AI, as exemplified by models like GPT-3 and GPT-4, is essentially altering the way we perceive and utilize AI. These models are versatile and flexible, capable of producing human-like text that's incredibly convincing. This has led to applications far beyond those envisioned with traditional machine learning paradigms. From generating creative content like poetry and articles to enhancing chatbots and virtual assistants, and even facilitating programming through code generation, generative AI is transforming the digital landscape. Furthermore, it's forcing us to grapple with new ethical and philosophical questions.
As these AI models become more integrated into our daily lives, we must navigate the challenges and opportunities they present, ultimately shaping the future of AI development and its role in our society. This new era of AI, marked by the rise of generative models, indicates a potential paradigm shift, reminding us of Kuhn's insightful analysis of scientific revolutions.
In "The Structure of Scientific Revolutions," philosopher Thomas Kuhn introduced the idea of paradigm shifts - fundamental changes in the basic concepts and experimental practices of a scientific discipline. Today, I'd like to explore whether we might be experiencing a similar paradigm shift within the field of artificial intelligence (AI), specifically in the emergence of generative AI models like GPT-3 and GPT-4.
Kuhn's Theory: An Overview
In Kuhn's view, scientific disciplines operate within paradigms - sets of practices and assumptions about the nature of the field. These paradigms guide the kinds of questions scientists ask and the methods they use to answer them. Over time, however, anomalies arise within the paradigm, results or phenomena that cannot be adequately explained. This leads to a state of crisis and, eventually, a paradigm shift or scientific revolution, where a new paradigm replaces the old one. Let's delve into these concepts a bit more deeply.
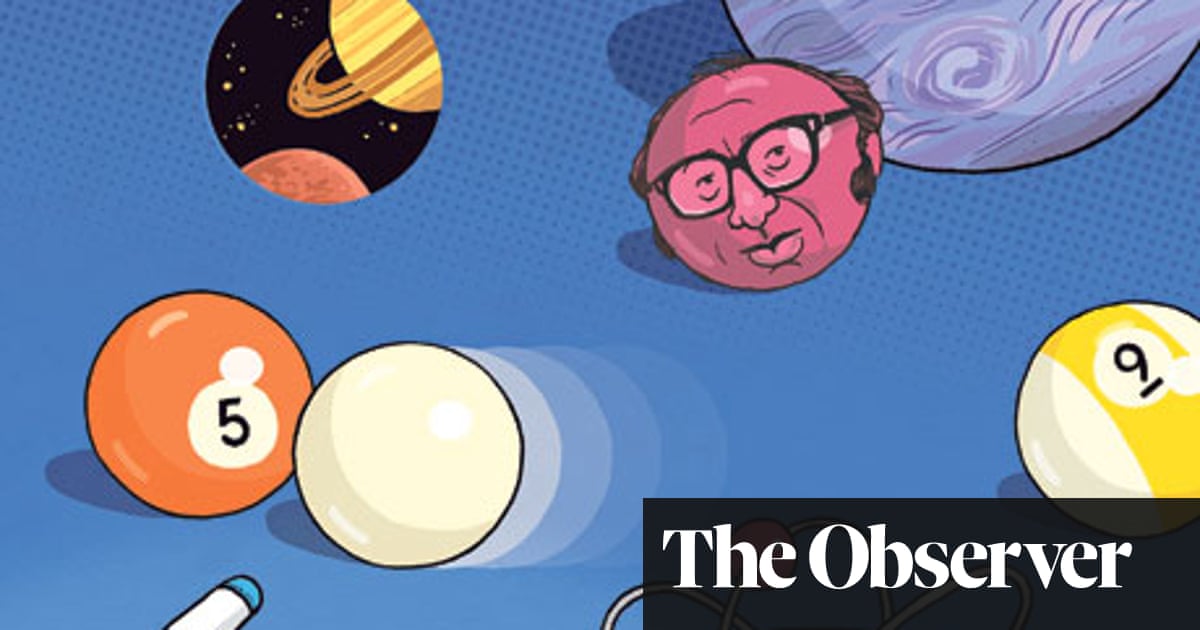
Paradigm Shift
A "paradigm shift" in the context of science is a fundamental change in the basic concepts and practices of a scientific discipline. According to Kuhn, scientific fields undergo periodic "paradigm shifts" rather than solely progressing in a linear, steady manner. Here are some historical examples of paradigm shifts:
- The Copernican Revolution: Before Copernicus, the Ptolemaic model, which proposed that the Earth was at the centre of the universe (geocentric), was the accepted paradigm. Copernicus, however, proposed a heliocentric model where the sun was the centre and the Earth revolved around it. This eventually resulted in a paradigm shift in astronomy and related fields.
- The Darwinian Revolution: Before Darwin, many believed in creationism or that species were immutable. Darwin's theory of evolution by natural selection proposed that species could evolve over time based on environmental pressures. This caused a major paradigm shift in biology.
- Quantum Mechanics: Classical physics, established by Newton, could explain most phenomena but struggled with certain aspects of the micro-world. Quantum mechanics introduced a new paradigm to understand the behaviour of particles at the atomic and subatomic levels, where the classical laws of physics do not hold.
- Plate Tectonics: For a long time, the Earth's continents were thought to be fixed in place. The theory of plate tectonics, developed in the mid-20th century, proposed that the Earth's crust is divided into large plates that slowly move. This caused a paradigm shift in earth sciences, changing our understanding of phenomena like earthquakes and volcanoes.
- The shift from Newtonian physics to Einstein's Theory of Relativity: Newton's laws of motion and universal gravitation were considered unchallenged until Einstein's theory of relativity came into the picture, proposing that the laws of physics are the same for all non-accelerating observers, the speed of light within a vacuum is the same no matter the speed at which an observer travels. This caused a significant shift in the field of physics.
Deep Dive into Kuhn's Work
To understand the potential paradigm shift in AI better, it helps to unpack Kuhn's work in more detail. His book is divided into several enlightening chapters that explore the progression of scientific understanding and innovation.
Kuhn introduces the concept of a scientific paradigm and sets the stage for his argument that science does not progress linearly but rather through a series of "revolutions" or paradigm shifts. He outlines how a paradigm is established, becoming "normal science." This is the routine work of scientists working within a paradigm, solving puzzles that the paradigm defines.
He further elaborates on "normal science," arguing that it involves puzzle-solving within the reigning paradigm and does not aim to discover new phenomena or theories. During periods of "normal science" scientists attempt to bring all phenomena in line with the established paradigm, often going to great lengths to account for anomalies or observations that do not fit the paradigm.
Kuhn emphasizes the overarching importance of the paradigm in shaping scientific thought and practice. It determines the standards and rules by which scientific work is conducted and judged. He introduces the concept of anomaly and how persistent anomalies can eventually undermine faith in the prevailing paradigm, leading to a state of "crisis" within the scientific community.
Further, Kuhn explains that the "crisis" phase occurs when the established paradigm fails to account for increasingly evident anomalies, leading to a period of scientific innovation and the development of new theories.
Kuhn delves into the various ways in which scientists respond to a crisis, ranging from ad hoc modifications of their theories to the complete rejection of existing paradigms. He describes how a scientific revolution culminates in the adoption of a new paradigm, which then becomes the basis for a new phase of normal science.
In the final chapter, Kuhn discusses how paradigm shifts represent fundamental changes in how scientists view the world, often involving changes in the very language and concepts they use.

Newer applications of ChatGPT / GPT-4
Here are some unusual applications of GPT-3.5 / GPT-4.
The versatility of generative AI models such as ChatGPT is truly astonishing and is being highlighted by their deployment in a variety of unexpected applications. A recent research experiment combined a brain scanner with a language model to provide insights into human thoughts. This marked an intersection between neuroscience and AI, demonstrating the model's potential in understanding and interpreting neural data.
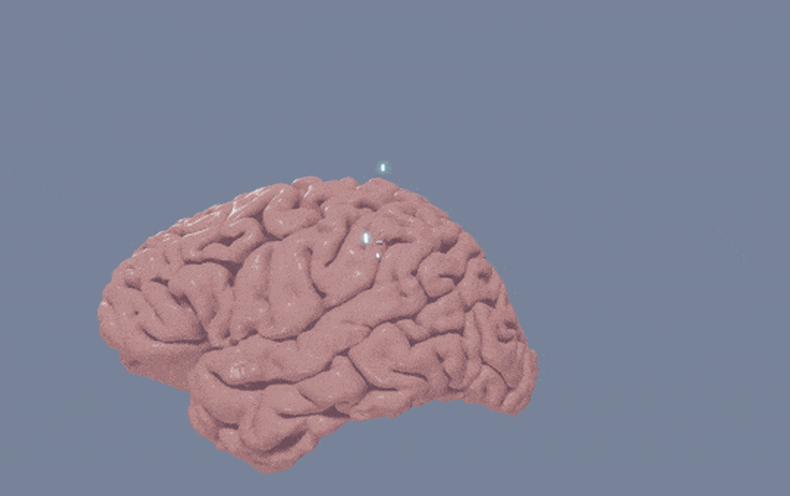
In another instance, ChatGPT was used for zero-shot analysis in predicting stock market movements, showcasing its potential in financial analytics and decision-making, a field previously dominated by complex quantitative models.
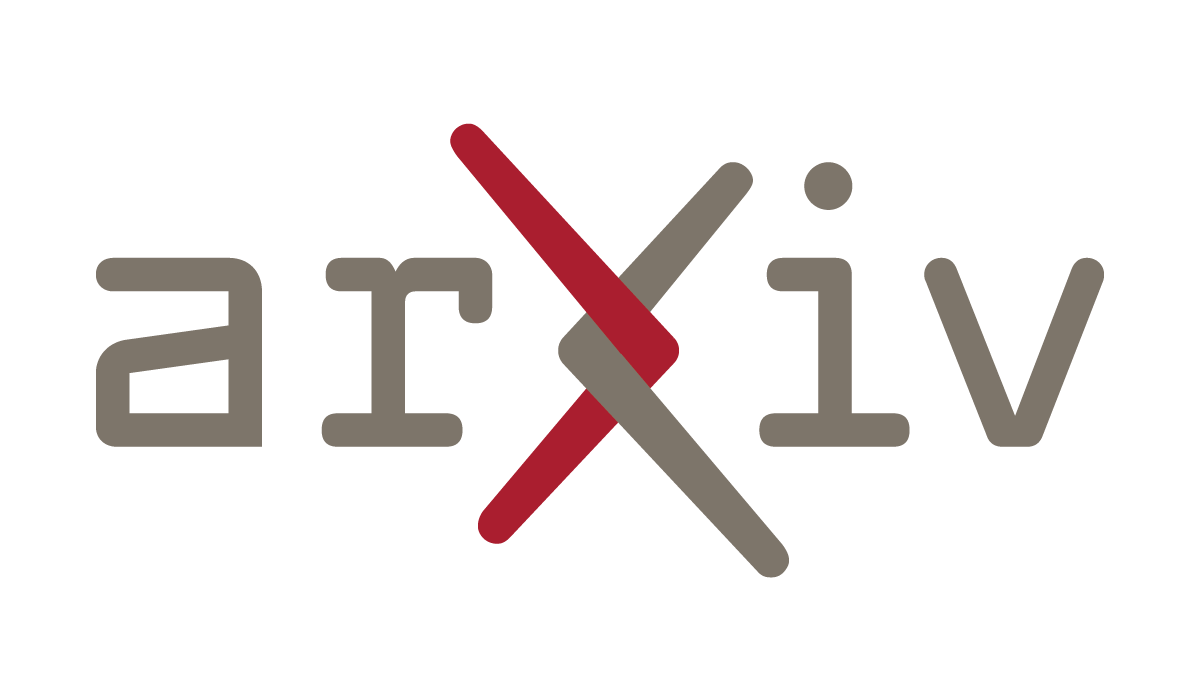
Finally, the possible use of ChatGPT in addressing global warming indicates its potential for societal and environmental issues.
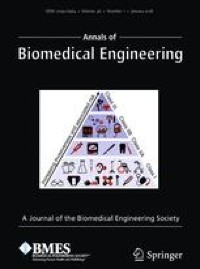
Generative AI through Kuhn's Lens
As we return to our examination of generative AI as a potential paradigm shift, Kuhn's analysis offers a useful framework. The rise of machine learning represents an example of "normal science" under Kuhn's model, with researchers working to solve specific problems within this paradigm. The current machine learning approach involves training models on large amounts of data, allowing them to learn patterns and make decisions based on those patterns. This has led to significant advancements in many areas, from image and speech recognition to natural language processing.
The current paradigm in AI research has encountered a few persistent anomalies. For one, deep learning models often require vast amounts of training data, and the data must be well-labelled. This presents problems of scalability and access, particularly for languages and communities with less available data. Furthermore, there's the "black box" problem of interpretability - it's often unclear why a model has made a particular decision, making it hard to trust the results. These persistent anomalies hint at a brewing crisis within the current AI paradigm.
The advent of generative AI and, more broadly, deep learning and artificial intelligence, can indeed be considered a paradigm shift in many ways, especially in the fields of computer science and technology, and potentially in many other disciplines such as healthcare, linguistics, arts, social sciences, and more.
Here's how this shift can be understood in the context of Kuhn's theory:
- Change in Basic Concepts and Experimental Practices: Traditionally, many computational and analytical tasks were based on manually designed algorithms with explicit rules. With the rise of deep learning, the paradigm shifted to algorithms that learn patterns from data. Generative AI models like GPT (Generative Pretrained Transformer) further expanded this by being capable of generating new content, not just analyzing existing data.
- Resolution of Anomalies: Traditional AI struggled with tasks that involved understanding complex patterns, like natural language processing or image recognition. Deep learning and generative AI provided a new way to approach these problems, outperforming older methods and offering solutions to previously challenging anomalies.
- Adoption by the Scientific Community: Over the past decade, the field has rapidly moved towards this new paradigm. Deep learning and generative AI models have become standard tools in computer science and many other fields.
- New Research Questions and Methods: This paradigm shift has opened up new areas of research, such as how to make AI models more interpretable, how to ensure their ethical use, and how to combine them with other AI techniques.
However, it's worth noting that whether something represents a paradigm shift can often only be fully assessed in retrospect. As AI and deep learning continue to evolve and mature, their status as a "paradigm shift" will likely become clearer. Also, as with all paradigm shifts, this transition has also brought along its own set of new challenges and questions about the implications and applications of these technologies.
In the current paradigm, scientists work on refining algorithms, optimizing architectures, and collecting and labelling vast datasets. Their work is guided by the belief that improved performance on benchmarks equates to progress in AI.
AI researchers in the current paradigm often try to account for anomalies by tweaking existing models or inventing new ones within the same fundamental framework. For instance, when models underperform in certain tasks, researchers often refine their architectures or gather more training data.
The dominance of machine learning in AI research has shaped the field's direction. It's set the standards and rules by which work is judged - for example, performance on standard benchmarks is often considered the ultimate measure of an algorithm's effectiveness.
However, Persistent anomalies in the current paradigm include the "black box" problem (the lack of interpretability in deep learning models) and the fact that models often require vast amounts of data to perform well. There's also the ethical issue of AI systems potentially amplifying existing biases.
The accumulation of these anomalies may be leading to a crisis in the AI field, where researchers increasingly recognize the limitations of the current paradigm. This is leading to the exploration of new theories and approaches.

In response to these anomalies, there have been efforts to modify existing models (ad hoc modifications) to better handle these problems. For instance, researchers have developed techniques to "explain" the decisions made by black box models or make them more transparent.
The rise of generative AI models like GPT-3 and GPT-4 might be seen as the beginning of a scientific revolution in the field of AI. These models represent a fundamentally different approach to AI, one that's more adaptable and flexible.
If generative AI is indeed causing a paradigm shift, this will likely change how researchers view AI. It might shift the focus from task-specific performance to versatility and adaptability. It might also lead to new ways of thinking about and dealing with the ethical implications of AI.
Moreover, the emergence of generative AI has brought with it a new set of questions and challenges that are shaping the field's research agenda. How do we ensure the ethical use of these powerful models? How can we make their outputs more understandable and controllable? How do we handle issues of bias in the data they're trained on? These are not merely technical questions but cut to the core of what we want and expect from AI. It's a different set of questions from those of the previous paradigm, indicative of a paradigm shift.
To sum up:
So, are we experiencing a paradigm shift in AI? According to Kuhn's theory, a paradigm shift is more than just the adoption of a new technology or technique; it's a fundamental reconfiguration of the field's guiding assumptions and methods. In this regard, the rise of generative AI models seems to fit the bill. They represent not just a new tool, but a different approach to AI - one that brings with it a new set of questions, challenges, and possibilities. However, I don't see these as paradigm shifts rather we have reached a crisis stage where new questions are being asked and anomalies are being addressed.
The above-mentioned applications of chatGPT published recently underscore the versatility and adaptability of generative AI. These are not mere incremental improvements over previous AI technologies; instead, they represent new ways of leveraging AI to solve problems, signalling a potential for a paradigm shift. It shows that we are moving away from narrow, task-specific AI towards more flexible, general-purpose models.
As Kuhn would predict, this shift isn't just about technology; it's also reshaping the kinds of questions we ask and the methodologies we use to answer them. This exploratory phase is indicative of a 'crisis' phase in Kuhn's model, which usually precedes a paradigm shift. It’s an exciting time to be witnessing and studying these changes, as they could redefine our understanding and application of AI.
I think this is the start of a revolution around the corner, which may soon lead to a paradigm change.
Research this week:
VisionLLM: Large Language Model is also an Open-Ended Decoder for Vision-Centric Tasks
The new VisionLLM framework offers a novel approach to vision-centric tasks, treating images as a foreign language, and allowing open-ended tasks with varying levels of customization through language instructions, achieving competitive results comparable to task-specific models, and setting a new baseline for generalist vision and language models.
TrueTeacher: Learning Factual Consistency Evaluation with Large Language Models
TrueTeacher, a novel method for generating synthetic data, outperforms existing models in evaluating summaries by annotating diverse model-generated summaries using a Large Language Model (LLM), proving superior in multilingual scenarios and offering enhanced robustness to domain-shift.
BioAug: Conditional Generation based Data Augmentation for Low-Resource Biomedical NER
BioAug, a novel data augmentation framework built on BART, effectively addresses data scarcity in Biomedical Named Entity Recognition (BioNER) by generating factual and diverse augmentations, leading to significant improvements in performance on benchmark datasets.
Drag Your GAN: Interactive Point-based Manipulation on the Generative Image Manifold
DragGAN, a newly proposed model, offers precise, user-interactive control over the manipulation of GAN-generated images, allowing for significant changes in pose, shape, expression, and layout across diverse categories, outperforming previous approaches in image manipulation and point tracking.
Events
Generative AI Unveiled - A Deep Dive into Language Models
We're excited to announce our next event, scheduled for May 27th. This session will continue our journey into AI, as we explore cutting-edge research, emerging technologies, and the future of AI in various industries. Don't miss this opportunity to learn from experts and connect with fellow AI enthusiasts.
In case you missed
Older posts that you may have missed
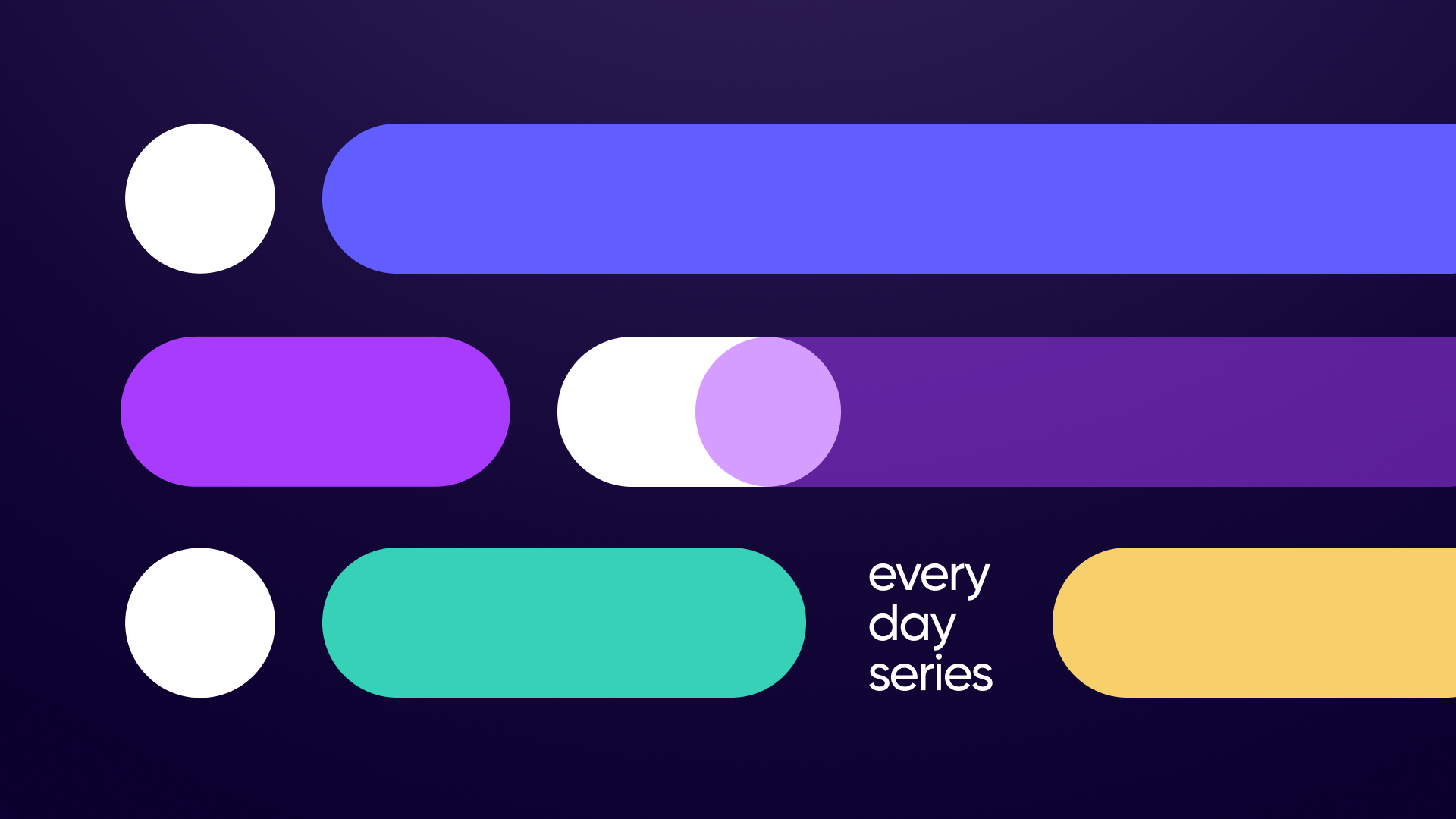

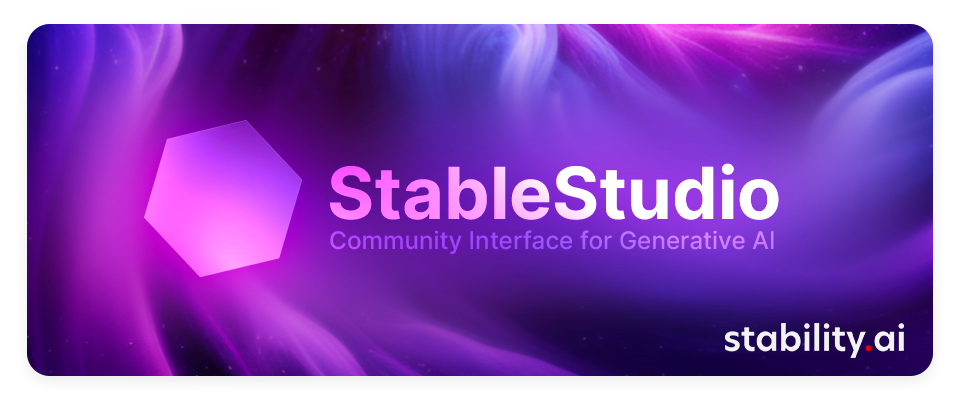

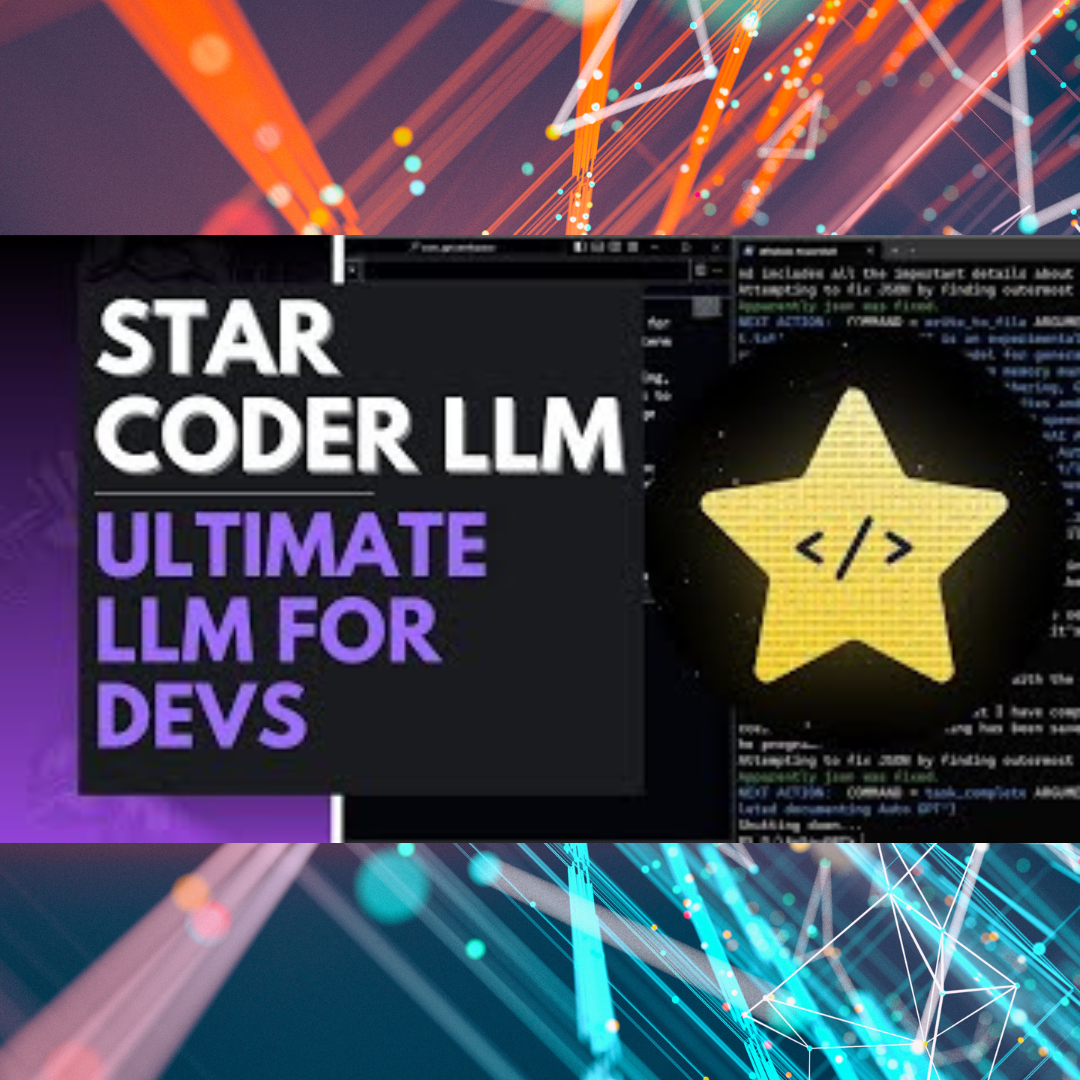



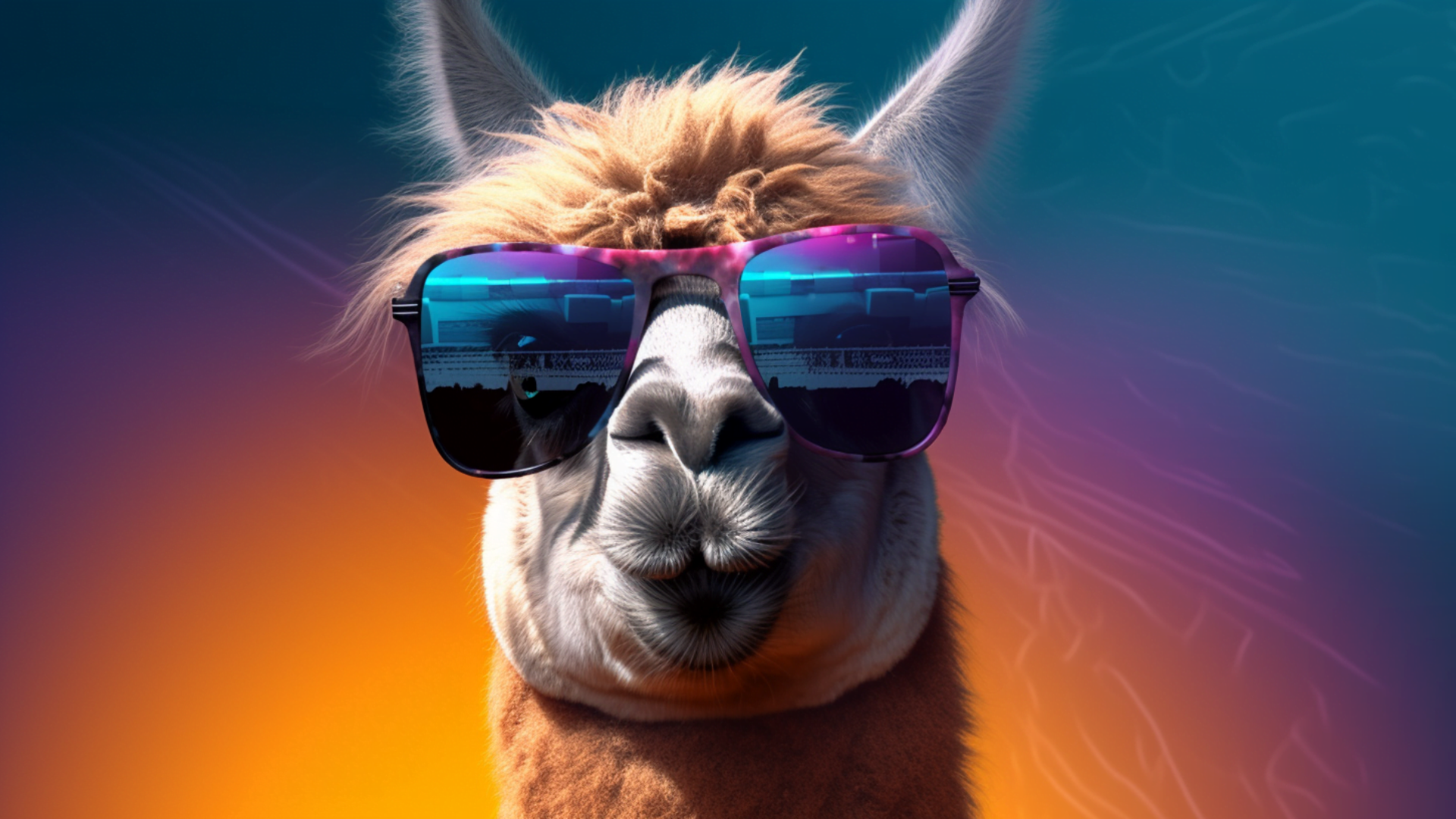


We research, curate and publish daily updates from the field of AI. Paid subscription gives you access to paid articles, a platform to build your own generative AI tools, invitations to closed events and open-source tools.
Consider becoming a paying subscriber to get the latest!
No spam, no sharing to third party. Only you and me.