Google's Tensor Processing Units (TPUs) have been at the forefront of accelerating artificial intelligence (AI) workloads since their introduction in 2016. The latest generation of TPUs, TPUs v4, takes things to a whole new level, delivering unprecedented levels of performance and efficiency that are poised to revolutionize the field of deep learning.
These specialized chips are designed to handle massive amounts of parallel processing, making them ideal for training and running large language models at the forefront of natural language processing (NLP) research.
The TPU v4 is a custom-built application-specific integrated circuit (ASIC) that has been optimized for performing tensor operations, which are the fundamental mathematical operations used in deep learning. With a single TPU v4 chip delivering up to 700 teraflops of computational power, this new hardware promises to revolutionize the field of NLP by enabling faster and more efficient model training. It enables:
- a nearly 10x leap forward in scaling ML system performance over TPU v3
- boosting energy efficiency ~2-3x compared to contemporary ML DSAs, and
- reducing CO2e as much as ~20x over these DSAs in typical on-premise data centres
The outstanding capabilities of TPU v4 make it the perfect choice for powering large language models due to its performance, scalability, efficiency, and availability. Delivering exascale machine learning performance, TPU v4 is equipped with 4096 chips connected through a cutting-edge optical circuit switch (OCS) designed in-house. The image below showcases an eighth of a TPU v4 pod.
Google's Cloud TPU v4 surpasses its predecessor, TPU v3, by delivering 2.1x higher performance per chip on average and boosting energy efficiency by 2.7x. Impressively, the average power consumption of a TPU v4 chip is a mere 200 watts.
TPU supercomputers are also at the forefront of innovation with hardware support for embeddings, an essential component of Deep Learning Recommendation Models (DLRMs). These models are widely used in various applications, such as advertising, search ranking, YouTube, and Google Play. Each TPU v4 is equipped with third-generation SparseCores, dataflow processors that enhance the performance of embedding-based models by 5x–7x, while only consuming 5% of the die area and power.
The potential applications of TPUs v4 are vast, spanning a wide range of industries and use cases. In healthcare, TPUs v4 could be used to train deep learning models that can diagnose diseases more accurately and efficiently than human doctors. In finance, TPUs v4 could be used to train fraud detection models that can identify fraudulent transactions in real-time, helping to prevent financial losses. And in autonomous vehicles, TPUs v4 could be used to train computer vision models that enable self-driving cars to detect and respond to their surroundings in real-time, ensuring safe and efficient transportation.
TPU v4 represents a major leap forward in the field of natural language processing, delivering unprecedented levels of performance and efficiency that are poised to revolutionize the way we approach large language model training. The exceptional performance, scalability, and availability of TPU supercomputers make them the go-to choice for powering large language models such as LaMDA, MUM, and PaLM. The impressive 540 billion-parameter PaLM model maintained a striking 57.8% of the peak hardware floating point performance for 50 days during training on TPU v4 supercomputers. The scalable interconnect of TPU v4 plays a crucial role in unlocking multidimensional model-partitioning techniques, which provide low-latency, high-throughput inference for these language models.
With their ability to train large-scale models faster and more efficiently, TPUs v4 are opening up new possibilities for NLP across a wide range of industries and use cases.
Read more:
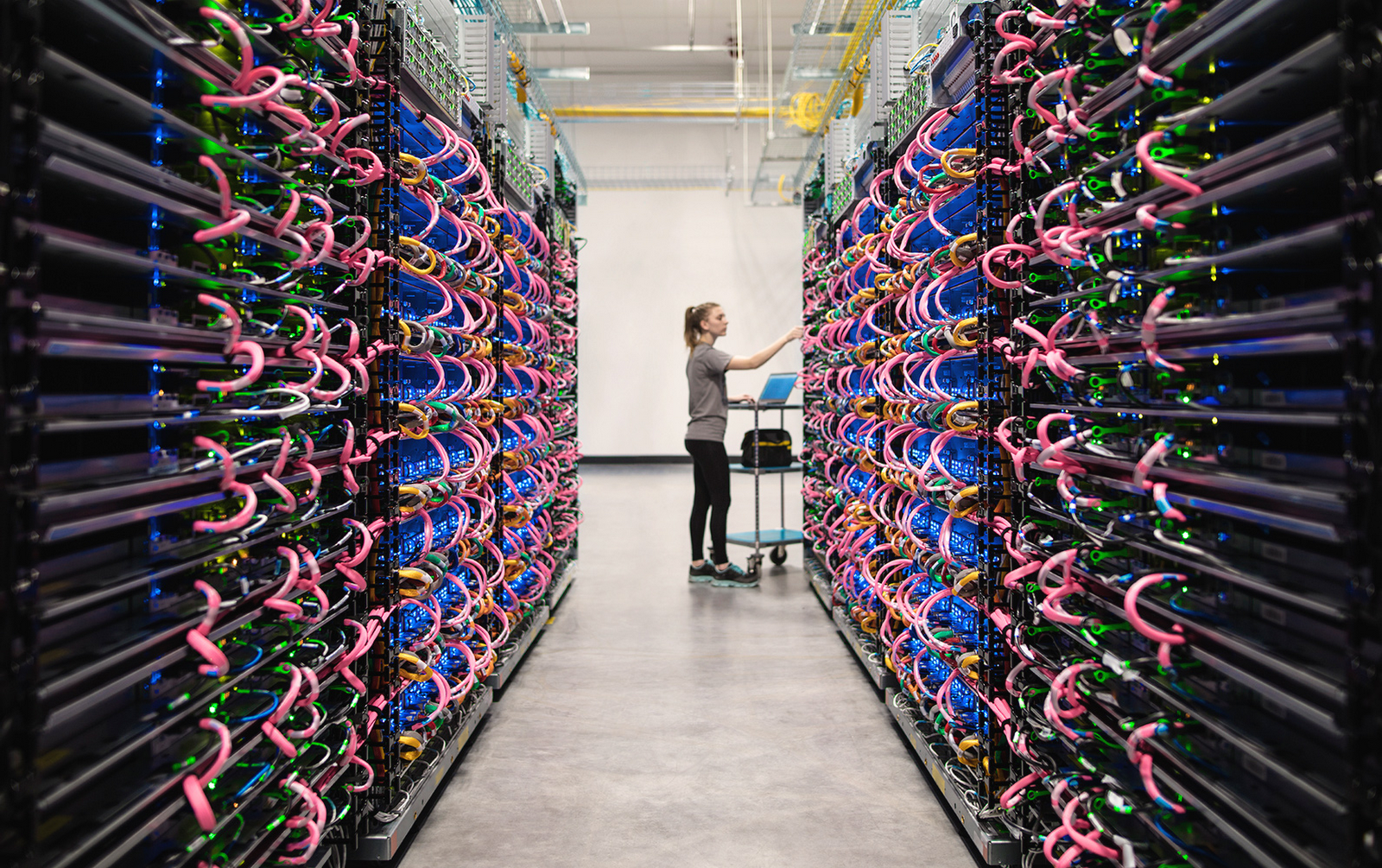
We research, curate and publish daily updates from the field of AI. Paid subscription gives you access to paid articles, a platform to build your own generative AI tools, invitations to closed events and open-source tools.
Consider becoming a paying subscriber to get the latest!