Recent advances in text-to-image generation have allowed for the creation of high-quality images from textual descriptions. However, it has been difficult to control the layout and shapes of objects in the image in a detailed and precise manner. Previous methods relied on pre-defined labels, limiting the flexibility of the generated images. To overcome this, a new method called SpaText has been developed.
This method allows for open-vocabulary scene control, meaning users can provide a natural language description of each region in the image they want to control. The method uses a novel spatio-textual representation based on a large-scale text-to-image dataset and is effective on two state-of-the-art image generation models.
The method also includes an alternative accelerated inference algorithm and automatic evaluation metrics. The evaluation shows that SpaText achieves state-of-the-art results in generating high-quality images with free-form textual scene control.
The aim is to provide the user with more control over the generated image by allowing them to provide a segmentation map with local text prompts, in addition to a global text prompt. However, current large-scale text-to-image datasets do not contain local text descriptions for each segment in the images.
To overcome this, a pre-trained panoptic segmentation model and a CLIP model are used to extract the objects in the image along with their textual descriptions. During training, random segments of the image are extracted, pre-processed, and their CLIP image embeddings are used to form the spatio-textual representation.
During inference, the local prompts are embedded into the CLIP text embedding space, converted using the prior model to the CLIP image embeddings space, and stacked in the same shapes of the input masks to form the spatio-textual representation. This method allows for more fine-grained control over the generated image by enabling the user to provide detailed descriptions of each segment.
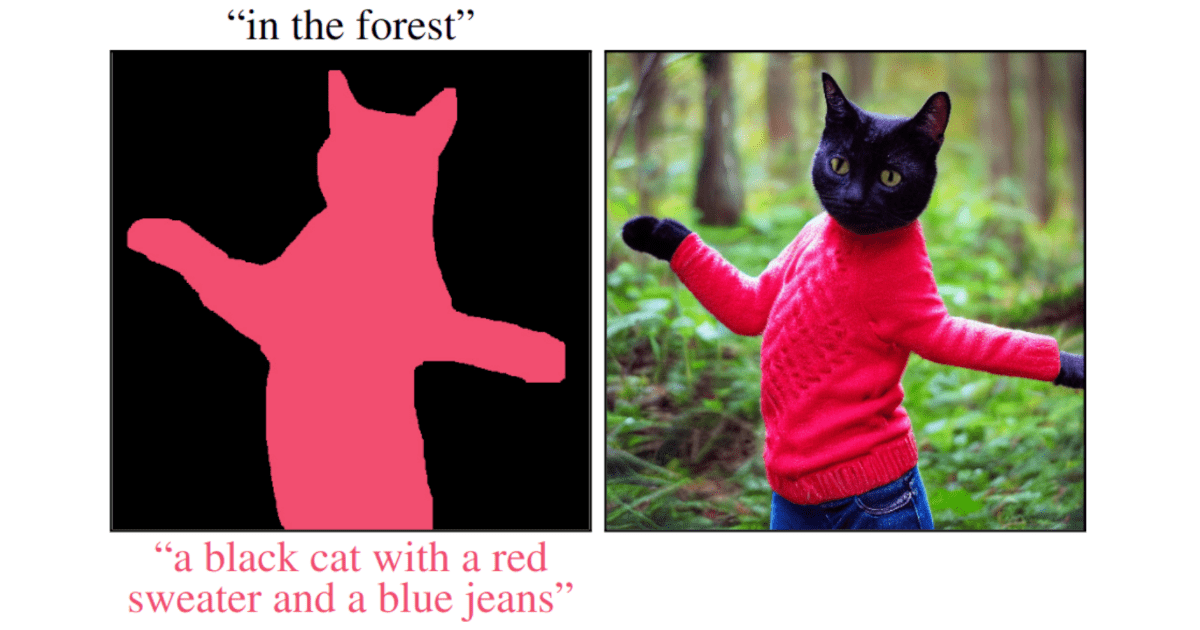
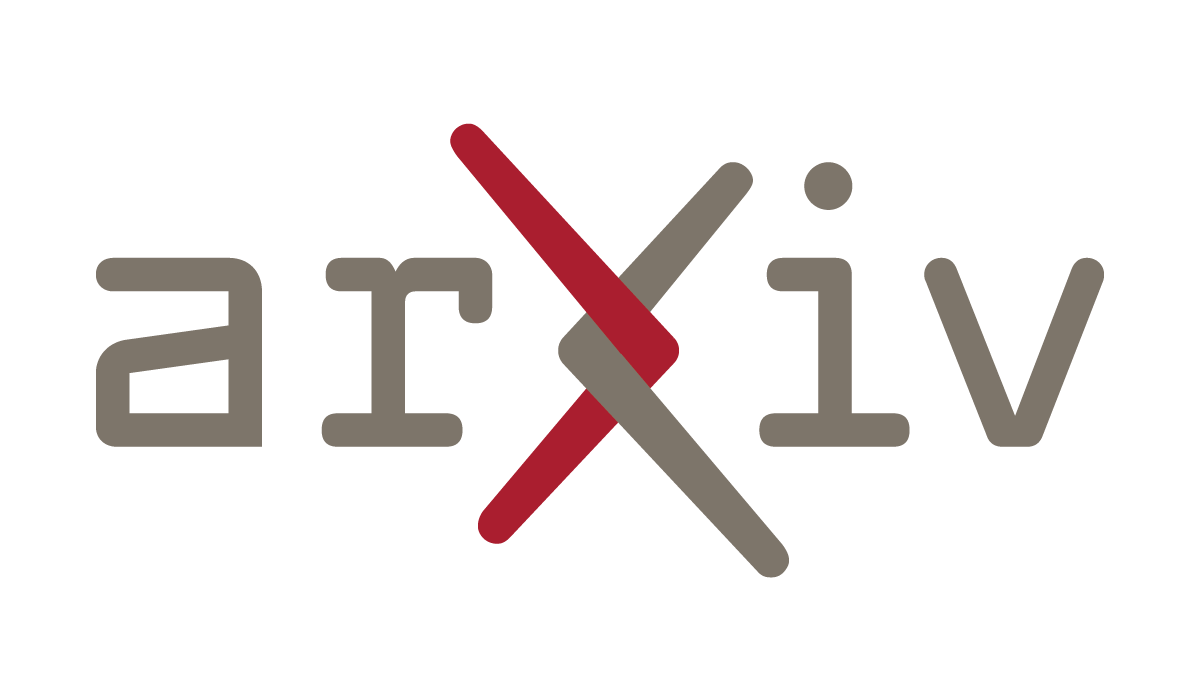
We research, curate and publish daily updates from the field of AI.
Consider becoming a paying subscriber to get the latest!