Text-to-image diffusion models have been gaining popularity due to their ability to generate stunning images from natural language descriptions. These models can rival the work of professional artists and photographers, but they are computationally expensive and slow to run. This is because they have complex network architectures and require tens of denoising iterations. As a result, high-end GPUs and cloud-based inference are required to run diffusion models at scale, which can be costly and have privacy implications.
However, researchers have recently developed a new approach that unlocks running text-to-image diffusion models on mobile devices in less than 2 seconds. The approach, called SnapFusion, introduces an efficient network architecture and improves step distillation. Specifically, the researchers propose an efficient UNet by identifying the redundancy of the original model and reducing the computation of the image decoder via data distillation. They also enhance step distillation by exploring training strategies and introducing regularization from classifier-free guidance.
Comparison w/ Stable Diffusion v1.5 on MS-COCO 2014 validation set (30K samples)

Image Credit: Github
The researchers conducted extensive experiments on MS-COCO and found that their model with 8 denoising steps achieves better FID and CLIP scores than Stable Diffusion v1.5 with 50 steps. This is an impressive result that demonstrates the effectiveness of their approach.
SnapFusion not only makes it possible to run text-to-image diffusion models on mobile devices, but it also democratizes content creation by bringing powerful text-to-image diffusion models to the hands of users. This could have significant implications for a wide range of applications, from social media to e-commerce.
On-Device Demo for SnapFusion
Since the publication of the SnapFusion paper, there have been some developments in the field of text-to-image synthesis. For example, researchers at OpenAI have developed DALL-E 2, a new version of their text-to-image synthesis model that is capable of generating images from more complex natural language descriptions. DALL-E 2 was trained on a dataset that includes text descriptions of objects in various settings and can generate images that include multiple objects and background elements.
Text-to-image diffusion models have the potential to transform content creation, but their computational demands have made them inaccessible to many users. SnapFusion represents a significant step forward in making these models more widely available by enabling them to run on mobile devices. As the field continues to advance, we can expect to see even more impressive results from text-to-image synthesis models like SnapFusion and DALL-E 2.
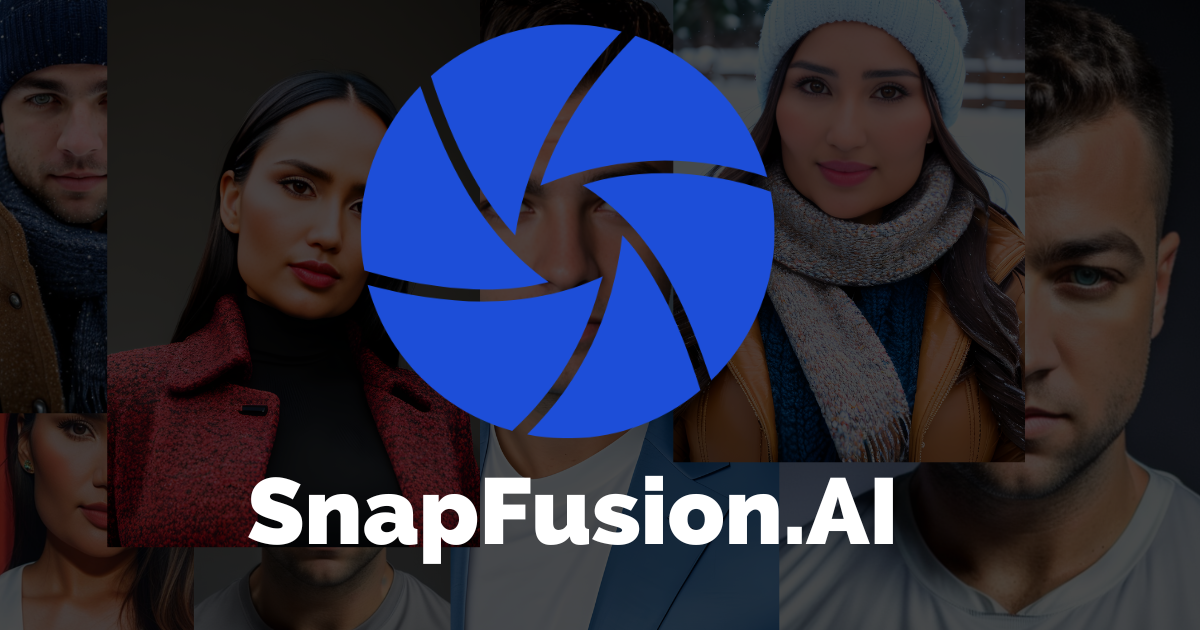

We research, curate and publish daily updates from the field of AI. Paid subscription gives you access to paid articles, a platform to build your own generative AI tools, invitations to closed events and open-source tools.
Consider becoming a paying subscriber to get the latest!