Empowering Ethical AI Through Transparency and Accountability
Have you ever wondered just how transparent and accountable artificial intelligence (AI) models are? As AI continues to influence our daily lives, from virtual assistants to recommendation systems, understanding the inner workings and potential biases of these models is crucial.
Fortunately, researchers at Stanford University's Institute for Human-Centered Artificial Intelligence (HAI) have introduced the Foundation Model Transparency Index, a groundbreaking initiative that sheds light on AI's previously hidden corners.
In this blog post, we'll explore the important points surrounding the Foundation Model Transparency Index and its significance in the world of AI ethics and accountability.
The Foundation Model Transparency Index: An Innovative Tool in AI
The Foundation Model Transparency Index is a comprehensive framework designed to assess and evaluate the transparency of large-scale AI models like GPT-3, BERT, and others. Transparency in this context refers to the clarity and openness with which AI models operate, revealing their decision-making processes and potential biases.
Why Transparency Matters
Transparency is crucial because it addresses long-standing concerns in the digital technology landscape. Deceptive ads, unclear pricing, and other transparency issues have plagued consumers for years. As commercial foundation models become more prevalent, we face similar threats to consumer protection. Furthermore, transparency in these models is vital for AI policy and empowering both industry and academic users to make informed decisions when working with these models
To assess transparency, Bommasani and CRFM Director Percy Liang assembled a multidisciplinary team from Stanford, MIT, and Princeton, collaborating to design a comprehensive scoring system known as the Foundation Model Transparency Index (FMTI).
This innovative index evaluates 100 distinct aspects of transparency, encompassing the entire lifecycle of a foundation model, from its development to its downstream applications.
When the team applied the FMTI to assess 10 major foundation model companies, the results revealed ample room for improvement. The highest scores, ranging from 47 to 54, offered no reason for celebration, while the lowest score hit a mere 12. These scores provide a clear benchmark for comparing these companies and, it is hoped, will serve as a catalyst for enhanced transparency efforts within the industry.
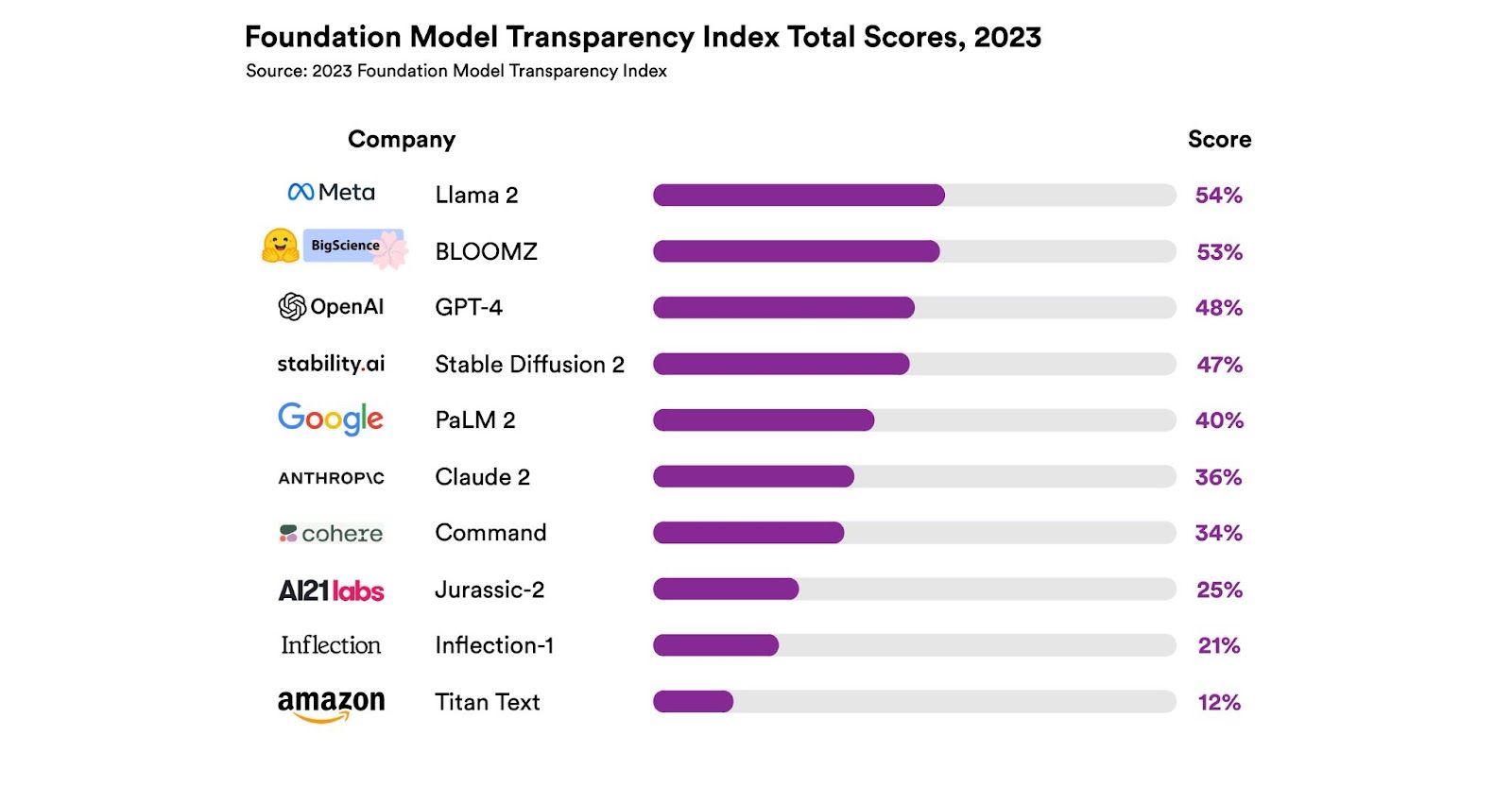
Key highlights of the Foundation Model Transparency Index include:
- Evaluation Metrics: The index employs a range of metrics to measure AI model transparency, such as explainability, fairness, and accountability. These metrics provide a holistic view of a model's strengths and weaknesses.
- Benchmarks: Researchers have established benchmarks that AI developers can use to assess and improve their models' transparency. These benchmarks set a new standard for accountability in the AI community.
- Real-World Impact: Understanding the transparency of AI models is crucial for identifying potential biases and ethical concerns, especially in applications like finance, healthcare, and criminal justice.
In the creation of the FMTI, Bommasani and his team meticulously developed a comprehensive set of 100 transparency indicators.
These indicators drew inspiration from both the AI literature and the well-established practices in the realm of social media, which boasts a more mature framework for safeguarding consumer interests.
The criteria were categorized into three key aspects: roughly a third of them pertain to the foundation model development process, covering critical details such as training data, labor inputs, and computational resources.
Another third revolves around the model itself, assessing its capabilities, trustworthiness, associated risks, and measures to mitigate those risks. The final third focuses on downstream use, encompassing aspects like disclosure of company policies regarding model distribution, safeguarding user data, model behavior, and the provision of avenues for feedback and recourse for affected individuals.

Image source:https://hai.stanford.edu/news/introducing-foundation-model-transparency-index
Implications for AI Ethics and Accountability
The introduction of the Foundation Model Transparency Index carries significant implications for AI ethics and accountability:
- Bias Mitigation: By identifying transparency issues, developers can take steps to mitigate biases and enhance fairness in AI systems, reducing the risk of perpetuating harmful stereotypes.
- Regulatory Compliance: As governments and regulatory bodies increasingly focus on AI ethics, the index can serve as a tool for organizations to ensure compliance with emerging AI regulations.
- Trust Building: Transparent AI models can foster trust among users and stakeholders, making AI technologies more acceptable and reliable.
Future Prospects
The Foundation Model Transparency Index is just the beginning of a broader conversation about AI ethics and accountability. As researchers continue to refine the index and AI developers strive for greater transparency, we can expect to see significant advancements in AI technology that align with our ethical and societal values.
the Foundation Model Transparency Index is a significant development in the world of AI ethics and accountability. It provides a much-needed framework for assessing and improving the transparency of AI models, paving the way for more responsible and trustworthy AI systems.
Read More to delve deeper into the Foundation Model Transparency Index and its implications:
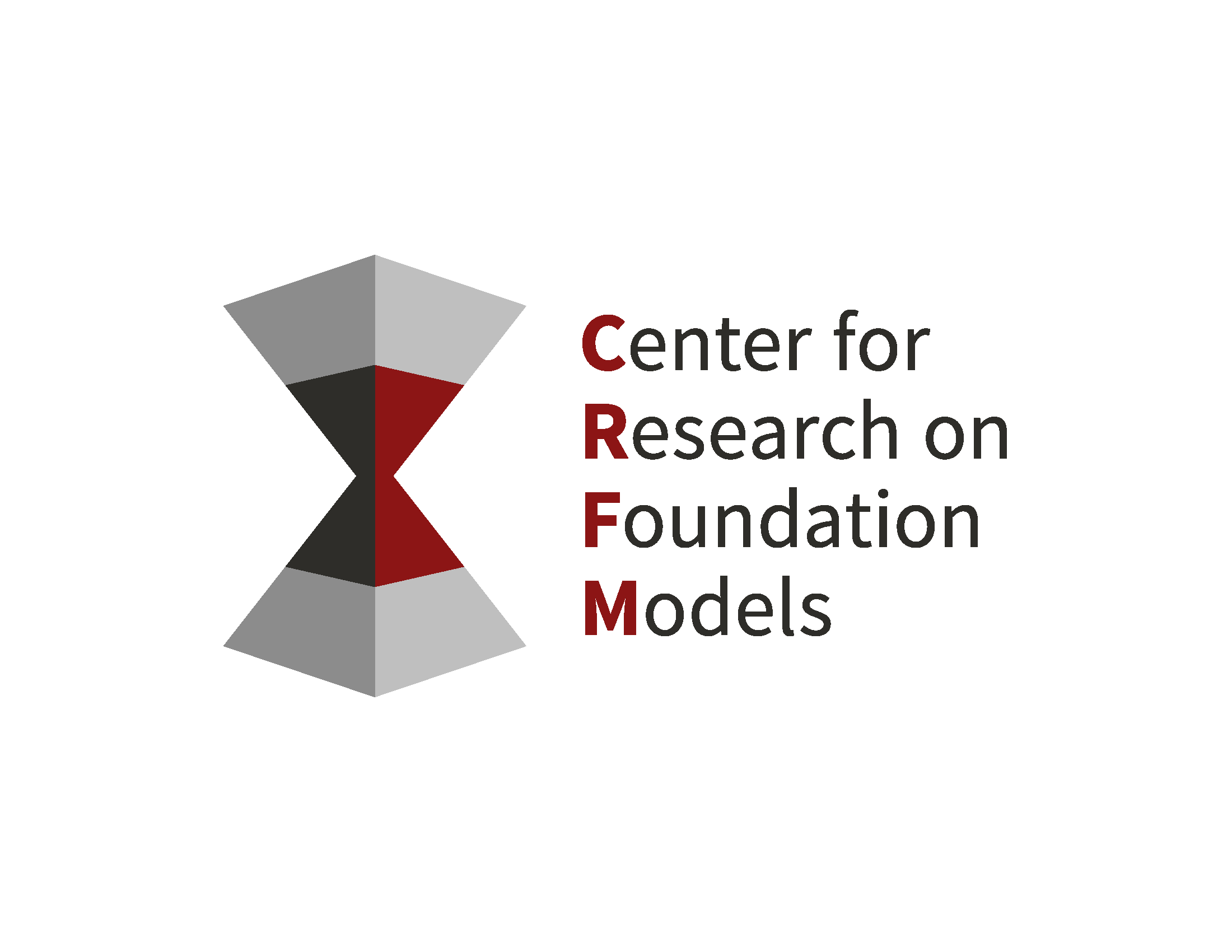
As AI becomes an integral part of our lives, understanding its transparency is vital for a more ethical and accountable AI future.
We research, curate, and publish daily updates from the field of AI. A paid subscription gives you access to paid articles, a platform to build your own generative AI tools, invitations to closed events, and open-source tools.
Consider becoming a paying subscriber to get the latest!