Aarushi beamed at the eager faces around her. "Did you know you can experiment with ChatGPT right on their website, using something called the Playground? It's like having your own laboratory to tweak and test how AI responds," she began, her voice filled with enthusiasm.
Model Size: The Powerhouse of Possibilities
"Let's start with the model size. Imagine this as choosing your Christmas tree. A larger tree – or model – can hold more decorations and lights, making it more impressive. But it also requires more effort to set up and more space in your living room. In the same way, larger LLMs can handle more complex tasks but need more computational power. It's about balancing grandeur with practicality."
Temperature: Crafting the Perfect Atmosphere
"Temperature in LLMs is like setting the mood for your Christmas party," Aarushi continued. "A lower temperature creates a familiar, cozy atmosphere, with traditional carols and well-loved recipes. But crank up the temperature, and you introduce the unexpected – maybe a surprise guest or an exotic dish. It's about how much novelty and creativity you want in your AI's responses."
"With a low temperature setting, let's say 0.2, the greeting might be traditional: 'Merry Christmas! May your holidays be filled with joy and peace.' But if we increase the temperature to 0.9, expect something more creative: 'Christmas Cheers! Wishing you a galaxy of stars and a season of delightful surprises!'"
Top-p and Top-k: Selecting the Best
"Top-p is like filling stockings with the best gifts based on each family member's personality," she explained. "You choose a variety of gifts, ensuring their total joy is maximized. Top-k, on the other hand, is like having Santa choose from the top three most wished-for gifts. Both methods ensure that the AI picks the most fitting words to continue the conversation."
"Now with top-p," she continued. "With a lower top-p, say 0.1, the model's response is more predictable: 'Happy Holidays from our family to yours!' But increase it to 0.9, and the model might generate something less conventional, like 'Jingle all the way on this festive day, where laughter and love light up the way!'"
"With top-k, let's set it to 3," Aarushi explained. "The model might give a standard response like, 'Wishing you a wonderful Christmas filled with happiness and fun.' But with a higher top-k, say 10, the AI could come up with something unique: 'May your Christmas be a jolly melody, with twinkling moments and cozy memories.'"
Number of Tokens: The Symphony of Words
"Regarding tokens," Aarushi added, "if we limit them, the model's response will be brief: 'Merry Christmas!' But with more tokens, it can elaborate: 'Merry Christmas! May the festive season bring you joy, laughter, and countless blessings.'"
"Tokens in an LLM are like the notes in a Christmas carol. Each one plays a small part in the larger melody. The number of tokens is like deciding the length of the carol. Too short, and the story is incomplete; too long, and it might lose its charm. The right number ensures the AI's response is just long enough to be engaging and informative."
Stop Sequences: Knowing When to Pause
"And with stop sequences, let's say we set it to stop after 'season's greetings,'" she said. "The AI will conclude its message accordingly: 'Season's greetings to you and your loved ones.' Without it, the model might continue: 'Season's greetings! Wishing you a new year filled with hope, happiness, and harmony.'"
"Stop sequences are like knowing when to end your Christmas dinner speech," Aarushi said with a smile. "You decide in advance when to conclude, like at the end of a sentimental note or a humorous anecdote. In LLMs, this helps to end the AI's response at the most appropriate moment, keeping it concise and relevant."
Repetition Penalty: The Variety of Christmas
"Lastly, repetition penalty," she noted. "At 1.0, the greeting might be repetitive: 'Merry Christmas, merry times, merry moments.' But at 1.2, it becomes more diverse: 'Merry Christmas, filled with festive cheer, warm gatherings, and joyful singing.'
"The repetition penalty ensures variety, just like you wouldn't serve the same Christmas meal every year. It encourages the AI to explore new words and ideas, keeping the conversation fresh and engaging. It's about striking the right balance between familiarity and innovation."
The villagers, now thoroughly intrigued, nodded in understanding. Aarushi's Christmas-themed analogies had turned a technical subject into an accessible and enjoyable learning experience, sparking a newfound interest in exploring the capabilities of LLMs.
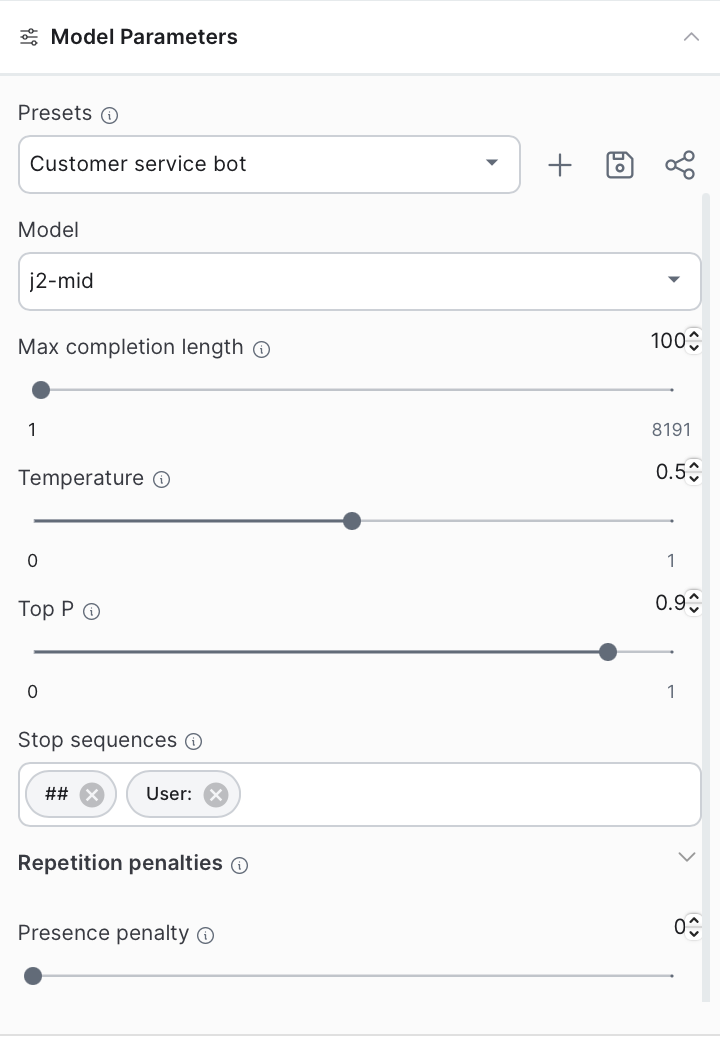
Charger: The Village Wiki and More
Eva stepped back onto the stage, her eyes shining with pride. "Thank you, Aarushi", she said. And now, we're thrilled to unveil our project, 'Charger' - a revolutionary AI tool designed specifically for our village."
"Charger is not just a wiki; it's a comprehensive AI system tailored to our village's needs," Eva began. "Imagine having a digital assistant that knows every nook and cranny of our village, every local event, every recipe from our community kitchen, and even stories from our elders."
Eva continued, "Charger gathers data from our village activities, local knowledge, and global databases. It then synthesizes this information into a Large Language Model, making it a unique and powerful tool. Whether you need advice on farming practices, local history, or even global news, Charger can provide accurate and contextually relevant information."
"Charger is designed to be user-friendly," Eva added. "It interacts in our local dialect, understands our customs, and even offers suggestions based on village trends. It's like having a friendly neighbor who's always there to help and advise."
"The applications are endless," Eva said with excitement. "Farmers can get real-time advice on weather and crops, students can access a wealth of educational materials, and anyone can get instant help with daily tasks. Charger can even assist in planning village events, keeping us all connected and informed."
"Think of Charger as a living library," Eva concluded. "It grows and learns with us, adapting to our needs and preserving our village's legacy for future generations. We envision Charger not just as a tool, but as a vital part of our community's growth and prosperity."
The villagers, mesmerized by the presentation, erupted into applause. The idea of Charger, an AI system deeply integrated with their daily lives and culture, sparked a sense of wonder and excitement.
As Eva was walking on the stage, Dr. Ingrid, Mo, the shopkeeper, and other audience exchanged impressed glances. Charger wasn't just a technological marvel; it was a testament to the village's innovative spirit and communal bond.
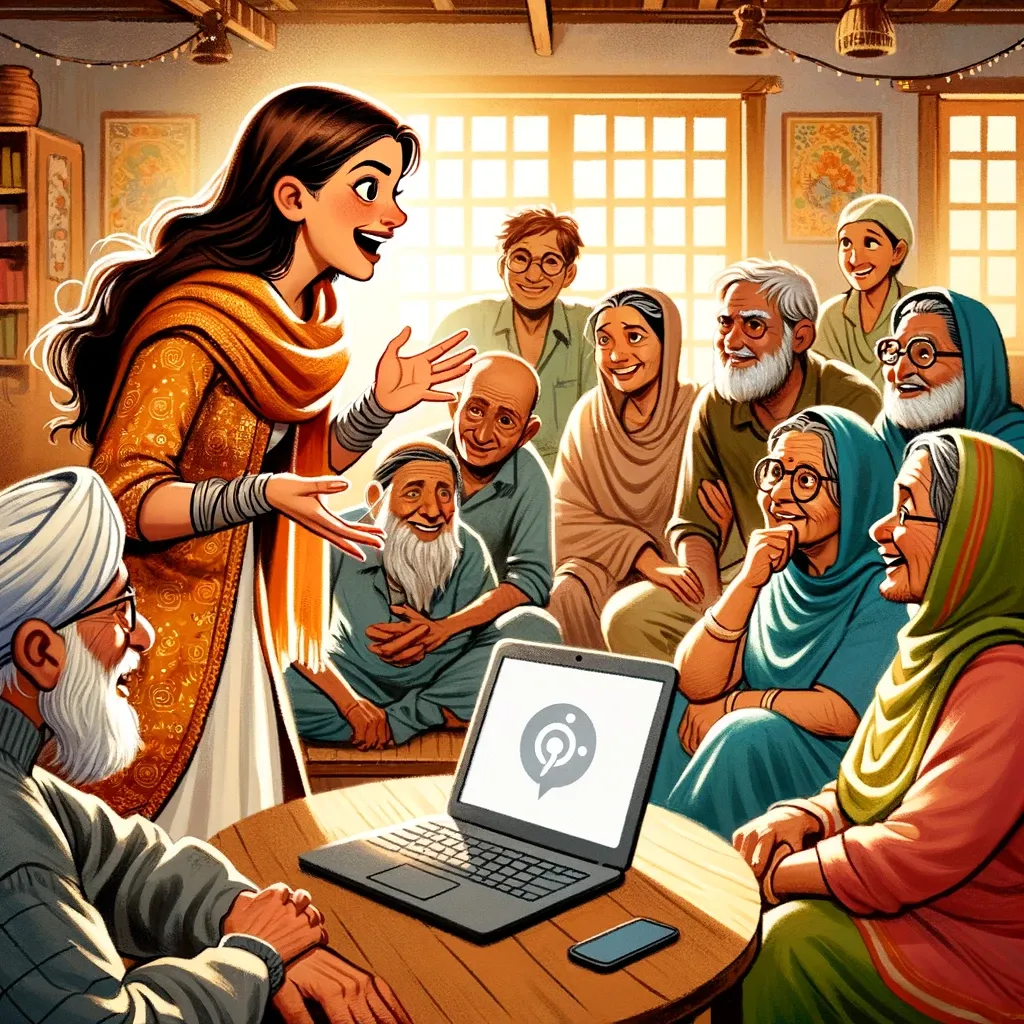
Technology Underneath
As the crowd settled, Eva took a deep breath, her eyes twinkling like the Christmas lights adorning the village hall. "Before I reveal Charger's secret recipe, let's take a moment to appreciate the journey we've embarked on," she began, her voice tinged with anticipation.
Setting the Stage
"Imagine a snowy Christmas Eve," Eva started, painting a vivid picture. "Our village, a canvas of white, each home a warm haven of yuletide joy. In this setting, we dreamed of something that could capture our village's spirit, its wisdom, its stories - and that's how Charger was born."
"The idea was simple yet ambitious," she continued. "To create an AI that wasn't just smart, but one that held our village's heart and soul. Think of Charger as a digital storyteller, a guardian of our traditions, ready to share our collective knowledge with the world."
"Every one of you has been a part of this," Eva said, her gaze sweeping over the audience. "Your stories, your knowledge, your questions - they've been the building blocks of Charger. It's like we've all been knitting a massive, colorful Christmas sweater, each thread representing our unique contributions."
"But we didn't stop there," Eva hinted. "We wanted Charger to not just reflect our village but to also have the wisdom of the world. It's like infusing our traditional Christmas recipes with flavors from across the globe, making them even more delightful."
"The result," Eva paused for effect, "is something truly magical. Charger is not just a tool; it's a reflection of us, our village, and our place in the wider world. But how did we make this magic happen? What's the secret ingredient that makes Charger so special?"
The audience leaned in, their curiosity piqued. Eva's words had painted a picture of a journey that was both deeply personal and ambitiously global. The excitement in the air was palpable as everyone awaited the revelation of Charger's secret.
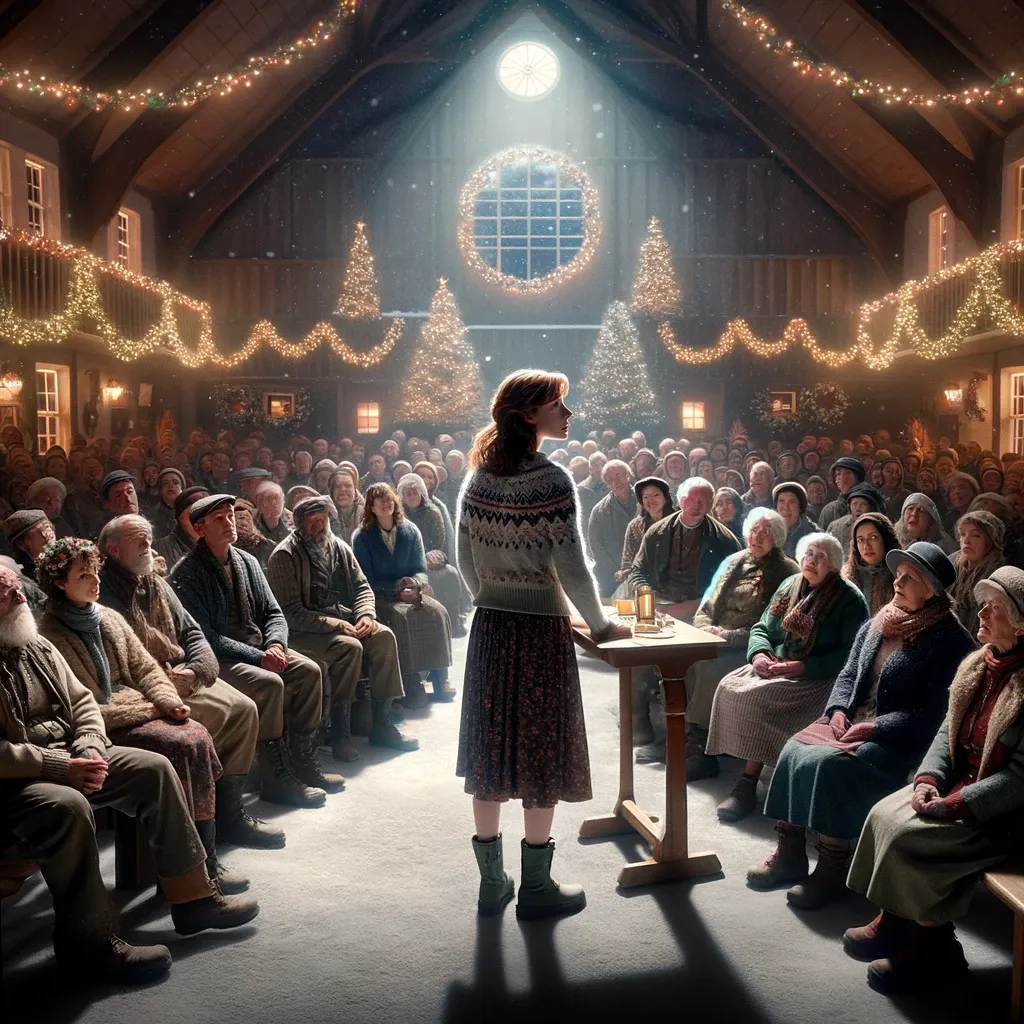
Transition to Technicalities
"And now," Eva said, her voice steady yet filled with excitement, "let's delve into the technical wizardry behind Charger. The first step in this magical journey was fine-tuning an existing foundational model..."
The crowd was fully engaged, hanging onto Eva's every word, eager to uncover the technical secrets that brought Charger to life.
Fine tuning a foundational model
Eva, standing confidently, delved deeper into Charger's creation process. "Let's talk about how we fine-tuned Charger, transforming it from a basic model into something uniquely ours, much like personalizing a Christmas cookie recipe with our special village ingredients. Imagine Charger as a Christmas cookie, and we're about to add our village's secret ingredients to make it uniquely ours."
The Essence of Fine-Tuning
"Fine-tuning Charger was like tweaking our traditional cookie recipe for the Christmas feast," Eva started. "We didn't just add new flavors; we altered the recipe to resonate with our village's taste. It's like infusing the dough with spices that tell stories of our village, ensuring that each bite reflects our community's essence."
"But what is fine tuning and why do we need it?"
"Fine-tuning is like giving Charger a specialized education," Eva explained. "It's the process where we take the basic structure of an AI model and teach it new tricks. Imagine teaching an old dog some new and fancy tricks specific to our village's needs. That's what we did with Charger – we enhanced its understanding to reflect our unique village life."
The Necessity of Fine-Tuning
"We opted for fine-tuning because Charger needed more than just basic knowledge," Eva continued. "Our village is unique, with its traditions, dialects, and local wisdom. To make Charger truly ours, it needed to learn these specifics, just like how a tailor fits a suit perfectly to its wearer. This level of customization is where fine-tuning shines, transforming Charger from a generic AI model to our village's personalized digital assistant."
Fine-Tuning vs. Prompt Engineering
Eva then contrasted fine-tuning with prompt engineering. "Think of prompt engineering as guiding Charger on what to say and how to say it based on external cues. But fine-tuning? It's about changing Charger from the inside out. It's a deeper, more intrinsic transformation, like embedding our village's heartbeat into Charger."
"Fine-tuning Charger wasn't simple," Eva admitted. "It's a sophisticated technique requiring a deep understanding of Large Language Models. It's like knowing exactly how to add the right amount of spice to a Christmas pudding so that it brings out the flavors perfectly. That's the level of expertise we applied to fine-tune Charger to understand and speak our village's language."
Eva, with a twinkle in her eye, turned to the different methods they employed to fine-tune Charger. "Fine-tuning Charger was like choosing the right technique to decorate our village Christmas tree. There are several ways to do it, each with its unique charm."
1. Feature-Based Fine-Tuning
"First, we have the feature-based fine-tuning," Eva began. "Imagine Charger as a Christmas tree and the pre-trained LLM as the undecorated tree. In this approach, we create decorations – or output embeddings – from our village data and use these to adorn our tree. The tree's core structure remains the same, but the decorations make it uniquely ours. This method is like adding a new star or some tinsel without changing the tree itself."
2. Updating The Output Layers
"The second approach is like adding a new set of lights to our tree," Eva continued. "We keep the main tree – the pre-trained LLM – unchanged but add new lights – the output layers – that we can control and adjust. It's a bit simpler than redesigning the whole tree but adds a noticeable new sparkle."
3. Updating All Layers
"Lastly, updating all layers is the most comprehensive method," Eva explained. "This is like revamping the entire Christmas tree – changing not just the decorations but reshaping the tree itself. It's a lot more work and requires more resources, but the result is a tree that's completely transformed and tailored to our village's taste. Updating all layers brings the best performance, but it's like preparing for the grandest Christmas feast; it takes a lot more effort and resources."
Eva, with an air of a seasoned storyteller, continued her deep dive into the world of fine-tuning. "Now, let's explore some more specialized techniques we tried with Charger - think of these as the high-tech tools we used to bring our Christmas tree to life in the most efficient way possible."
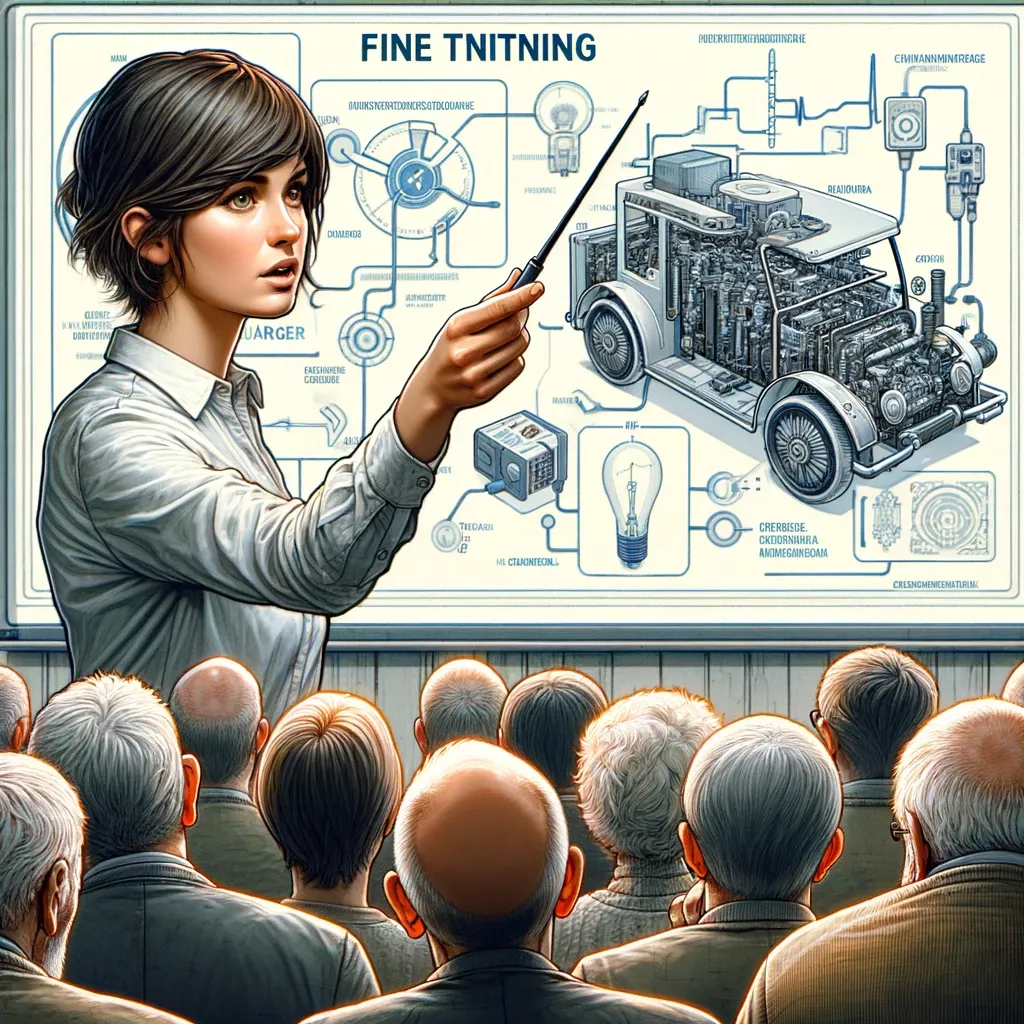
Parameter Efficient Fine-Tuning (PEFT)
"First up, PEFT, or Parameter Efficient Fine-Tuning," Eva said. "It reuses a pre-trained model to reduce computational and resource requirements. It's like using LED lights on our Christmas tree instead of the old bulky ones. They're efficient, use less power, and still make the tree look gorgeous. PEFT lets us tweak Charger without the heavy computational costs, fine-tuning just enough parameters to keep it accurate and efficient."
Prompt Tuning: The Art of Soft Prompts
"Then, we have prompt tuning," Eva continued. "Prompt tuning is a technique that sits at the intersection of prompt engineering and fine-tuning. It diverges from fine-tuning in that it doesn't alter the model's underlying parameters. What it does instead is incorporate prompt embeddings with each input given to the model. This process leads to the model itself revising the prompts, resulting in the creation of what are known as soft prompts."
"This technique is like having magical ornaments that change their design based on the songs we sing. In prompt tuning, we don't change Charger's core parameters. Instead, we add 'soft prompts' – these are like subtle hints to Charger, guiding it to respond in ways that reflect our village's unique character."
Low Rank Adaptation (LoRA)
"LoRA was another tool in our kit which we tried. It transforms weights into a lower-dimensional space, effectively reducing computational and storage demands" Eva added. "Imagine if we could reshape our Christmas ornaments into multiple designs without getting new ones. That's what LoRA does. It adapts certain parts of Charger, transforming its abilities without the need for extensive retraining or huge computational power."
Reinforcement Learning with Human Feedback (RLHF)
"And lastly, RLHF," Eva said, her voice filled with enthusiasm. "RLHF is a method where Charger learns directly from us, its users. It's like teaching someone to decorate a Christmas tree exactly how we like it, based on our feedback. In RLHF, Charger's education starts with a blend of supervised and reinforcement learning," Eva continued. "Supervised learning is like showing Charger pictures of how we traditionally celebrate Christmas, while reinforcement learning is about letting Charger try decorating the tree and then guiding it based on how well it does."
"The key to RLHF is human feedback," Eva pointed out. "We asked you all to interact with Charger, ranking or rating its responses, much like rating Christmas carols at our annual festival. This feedback is like a reward signal that tells Charger what it's doing right and where it needs to improve. These rankings and ratings are used to train a reward model," Eva explained. "It's like creating a guidebook for Charger, filled with our village's preferences and tastes. This reward model then helps Charger understand and adapt to what we, as a community, find most helpful and enjoyable."
"Not to mention that there are various techniques like Quantization which are used to make the process more efficient, but I am leaving that for some other conversation"
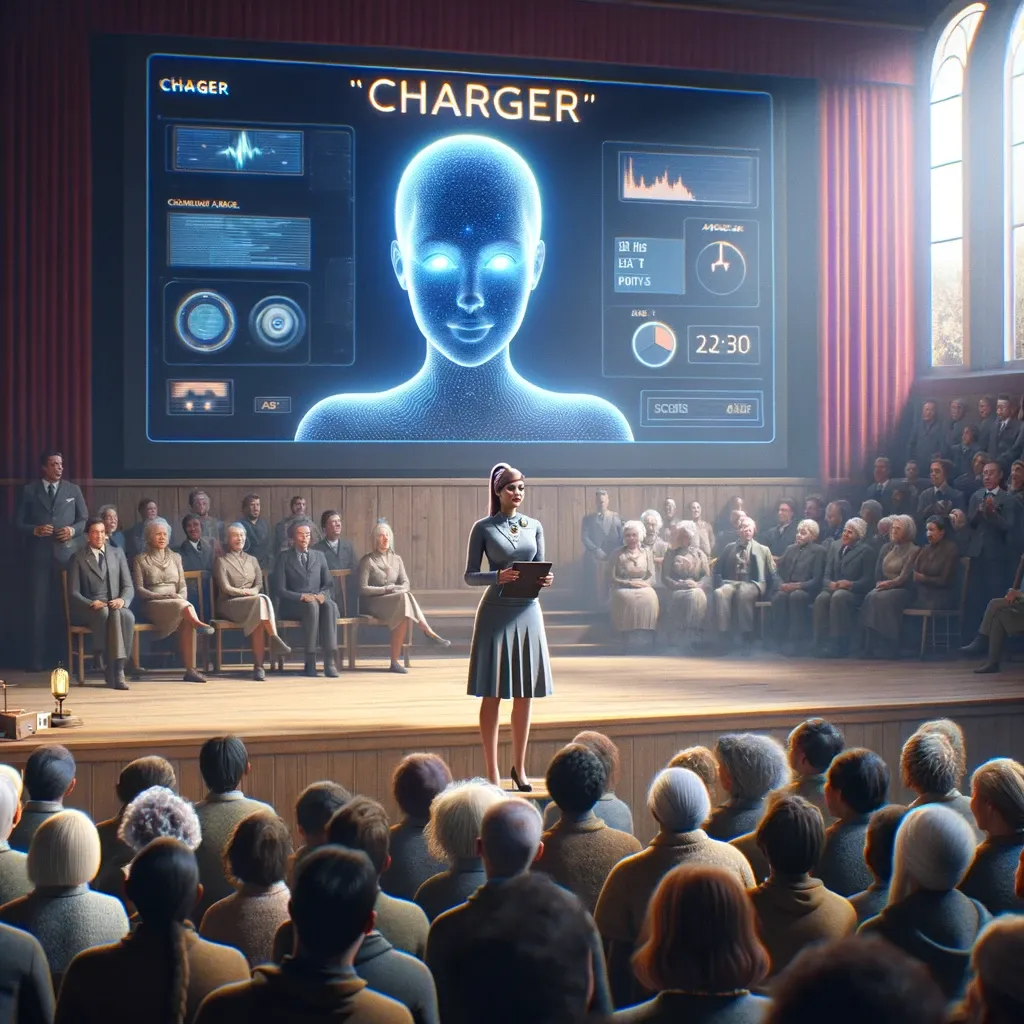
The villagers, now captivated by Eva's narrative, were seeing their Christmas tree in a new light – as a metaphor for the innovative AI technology that powered Charger. Each technique Eva described added another layer of understanding and appreciation for what they had achieved together as a community.
Mo, the inquisitive one, asked, "So, which method did you choose for Charger, Eva?"
Eva smiled. "We started with the feature-based approach, adding our unique village touch. But realizing we needed Charger to reflect our village more closely, we bravely ventured into RLHF. It was a challenging journey, but the results? Absolutely worth it!"
"It is the same way how ChatGPT or GPT-3.5 works. We collected a set of students, with the help of Dr. Silverman and Rohit and asked them to continuously rank and rate the responses generated by the foundational model. We then used this to train the reward model"
"The beauty of RLHF," Eva concluded, "is that it continuously molds Charger to fit our village's evolving needs. It's not just a one-time training; it's an ongoing process of learning and adapting, ensuring Charger remains an accurate and beloved part of our village life. We are also using it to flag inappropriate content generation by the tool and to some extent remove the bias from the AI model"
The villagers, now engrossed in the technicalities of Charger's development, applauded Eva's clear explanations. Her ability to translate complex AI concepts into relatable Christmas-themed analogies had made the world of fine-tuning both exciting and accessible.
Enjoyed unraveling the mysteries of AI with Everyday Stories? Share this gem with friends and family who'd love a jargon-free journey into the world of artificial intelligence!