Artificial intelligence (AI) and machine learning (ML) play significant roles in various industries, shaping the way we approach data-driven solutions. Within the AI/ML lifecycle, several stages, including data collection, preparation, training, evaluation, deployment, and monitoring, are integrated into the MLOps pipeline. However, as the transformative technology of Generative AI (GenAI) gains momentum, it necessitates distinct considerations and augmentations to ensure reproducibility, scalability, and responsible usage in the enterprise. In this article, we delve into the initial nuances required for adopting Generative AI in enterprise settings, while also contrasting it with Predictive AI.
Contrasting Generative AI and Predictive AI: Generative AI and Predictive AI are two distinct types of AI that serve different purposes. Generative AI involves the creation of new content, such as music, images, and texts, utilizing deep learning algorithms. It leverages large language models (LLMs) to exhibit emergent behavior and creativity. By training on extensive datasets encompassing general examples like Wikipedia and CommonCrawl, Generative AI generates novel instances resembling the training data.
This technology finds application in creative domains, facilitating the creation of artwork, music, and more. However, adopting Generative AI in an enterprise context demands a reproducible, scalable, and responsible approach to mitigate risks related to AI safety, misuse, and model robustness. The MLOps pipeline remains integral, albeit with specific nuances and augmentations tailored to Generative AI.
On the other hand, Predictive AI focuses on making predictions about future states or events, often employing supervised learning and historical training sets. By leveraging clustering, classification, and regression models, Predictive AI aids businesses in decision-making processes and customer behavior prediction. Training on historical data enables Predictive AI to make informed predictions based on patterns and trends.
Similar to Generative AI, the adoption of Predictive AI within an enterprise context necessitates a reproducible, scalable, and responsible approach to address AI safety, misuse, and model robustness concerns. The MLOps pipeline forms the foundation for implementing Predictive AI, albeit with its specific nuances and augmentations.
The Impact of Generative AI on Industries:
Generative AI has the potential to revolutionize various industries, including entertainment, advertising, design, and healthcare.
In the entertainment industry, Generative AI can aid in the creation of realistic CGI characters, special effects, and immersive virtual worlds.
Advertising agencies can utilize Generative AI to generate personalized content and targeted advertisements, enhancing customer engagement and conversion rates.
In design fields, Generative AI can assist architects, fashion designers, and product designers by generating novel designs and facilitating iterative design processes.
Healthcare can benefit from Generative AI by enabling the creation of synthetic medical images, drug discovery, and personalized treatment plans.
Ethical Considerations and Responsible Deployment:
With the increasing power of Generative AI, ethical considerations become paramount. Clear guidelines and regulations are necessary to prevent the misuse of generative models, such as deep fake videos and counterfeit products.
Responsible deployment of Generative AI involves transparency, fairness, and accountability. It is crucial to understand the biases present in the training data and ensure the generated content aligns with ethical standards.
Enterprises must adopt robust governance frameworks and establish guidelines for the ethical use of Generative AI, including strict data usage policies and comprehensive user consent procedures.
Overcoming Challenges in Generative AI Adoption:
Generative AI faces several challenges, including data quality, scalability, interpretability, and training stability.
Generating high-quality data for training generative models can be time-consuming and costly. Enterprises need to invest in data collection and curation processes to ensure the reliability and diversity of the training datasets.
Scaling generative models to handle large volumes of data and enable real-time generation requires powerful computing infrastructure and optimized algorithms.
Interpreting and explaining the decision-making process of generative models is a complex task, and efforts are being made to develop interpretability techniques specific to Generative AI.
Ensuring training stability is vital to prevent generative models from producing biased or misleading content. Regular monitoring and fine-tuning of models are necessary to maintain reliability and ethical standards.
Collaborative Approaches and Data Sharing:
Generative AI can benefit from collaborative approaches and data sharing within and across organizations.
Collaboration allows enterprises to access larger and more diverse datasets, which leads to improved model performance and generalization.
By establishing partnerships and sharing data, enterprises can collectively address the challenges of Generative AI adoption and advance the field responsibly.
Future Directions and Innovations:
The field of Generative AI is rapidly evolving, and ongoing research aims to address current limitations and unlock new possibilities.
Innovations such as conditional generative models, style transfer, and unsupervised learning techniques continue to push the boundaries of Generative AI.
Integrating Generative AI with other AI techniques, such as reinforcement learning and natural language processing, opens up opportunities for more sophisticated and interactive generative systems.
As the realms of Generative AI and Predictive AI continue to evolve, it is essential to understand their distinct characteristics and implications. Generative AI focuses on generating new content through deep learning algorithms, while Predictive AI centers around making predictions based on historical data. Adopting these technologies in an enterprise setting requires a reproducible, scalable, and responsible approach to address AI safety, misuse, and model robustness concerns.
The MLOps pipeline serves as the framework for integrating Generative AI and Predictive AI into existing workflows, with specific nuances and augmentations tailored to each. By navigating these nuances and leveraging the potential of both AI types, businesses can unlock transformative capabilities and drive innovation in diverse domains.
Read More
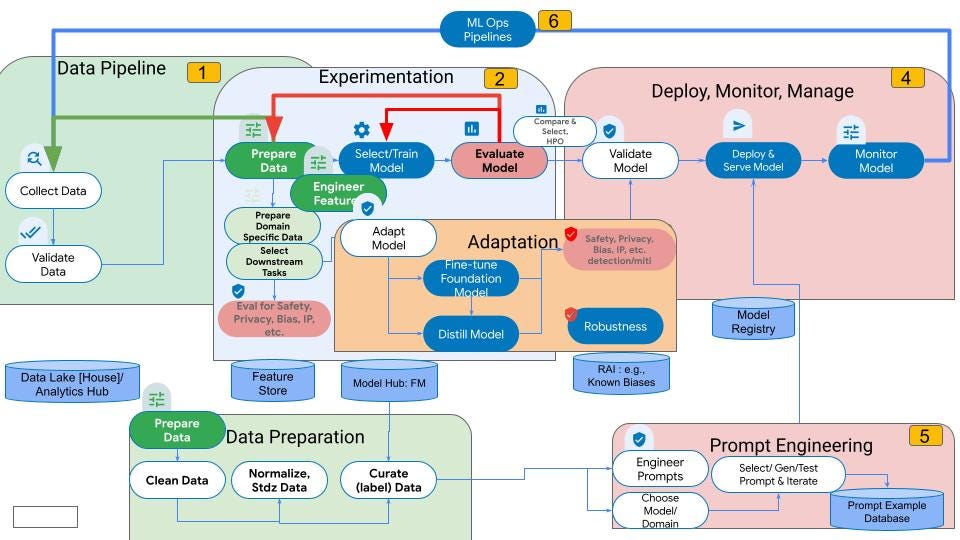
We research, curate and publish daily updates from the field of AI.
Consider becoming a paying subscriber to get the latest!