Fun with FunQA: Elevating Video Reasoning to New Heights
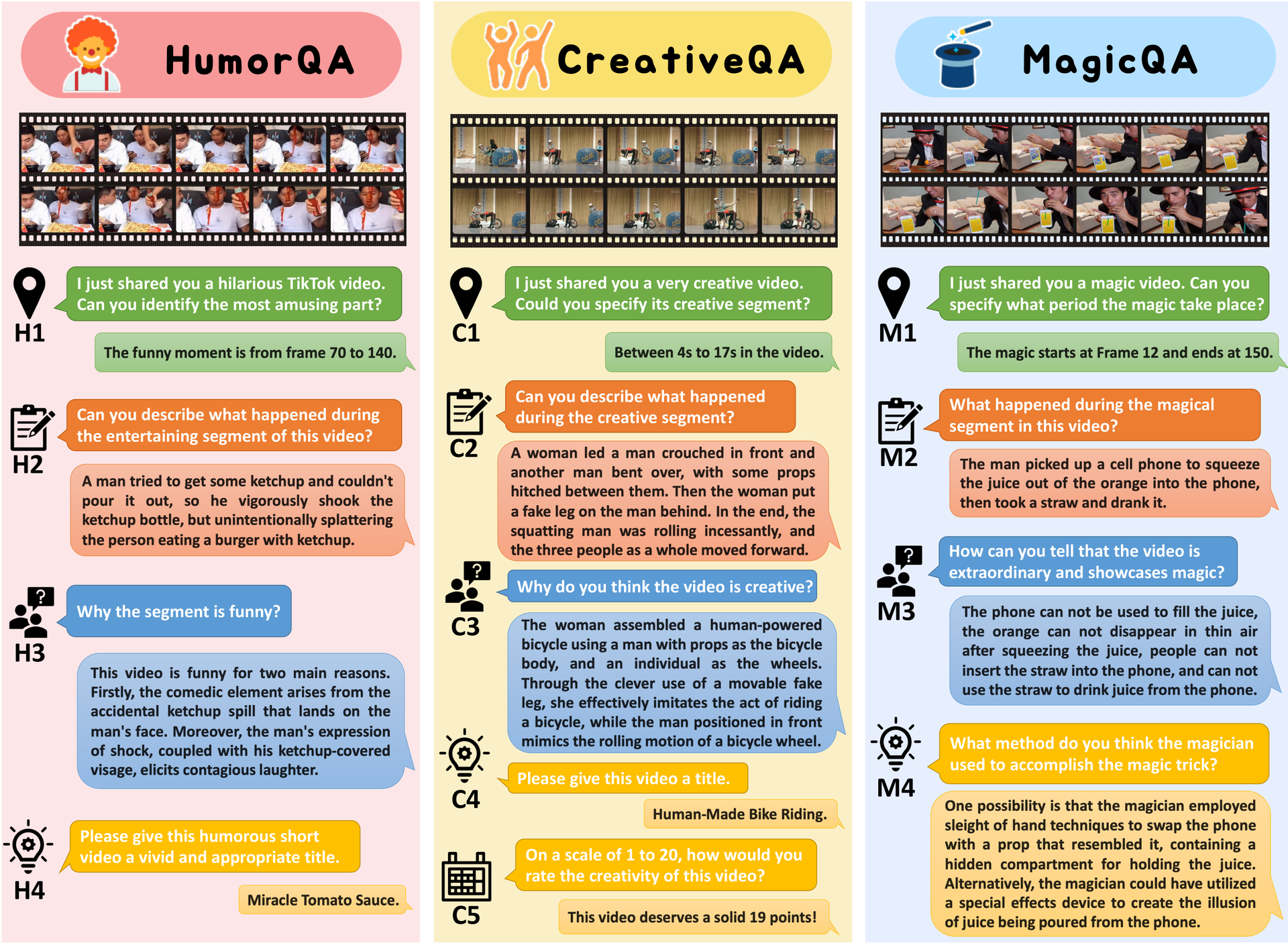
In the vast landscape of online content, it's no secret that surprising videos hold a special place in our hearts. From hilarious clips that tickle our funny bones to awe-inspiring performances and mind-bending visual illusions, these videos captivate our attention like few others. But have you ever wondered what makes them so enjoyable? It turns out that our fascination with these videos goes beyond mere visual stimuli. It's rooted in our innate ability to grasp and appreciate the commonsense violations depicted in these captivating moments.
Enter FunQA, a groundbreaking video question-answering (QA) dataset designed to push the boundaries of video reasoning by focusing on counter-intuitive and fun videos. Unlike conventional video QA benchmarks that often revolve around everyday contexts like cooking or instructional videos, FunQA ventures into uncharted territory, exploring three exciting and previously unexplored categories: HumorQA, CreativeQA, and MagicQA. Each subset presents unique challenges and demands, evaluating the depth of a model's video reasoning capabilities when confronted with counter-intuitiveness.
For every subset, FunQA sets forth rigorous QA tasks meticulously crafted to assess a model's proficiency in several dimensions. These tasks include counter-intuitive timestamp localization, providing detailed video descriptions, and reasoning around the counter-intuitive aspects of the videos. But the benchmark doesn't stop there. It also presents higher-level tasks that push the boundaries of video understanding, such as assigning a fitting and vivid title to the video and scoring its creativity.
In total, the FunQA benchmark boasts an impressive collection of 312,000 free-text QA pairs derived from 4,300 video clips, spanning an astonishing 24 hours of video content. The sheer scale and diversity of this dataset ensure that models will be thoroughly tested and evaluated across a wide range of scenarios.
To assess existing VideoQA models, extensive experiments have been conducted with the FunQA dataset. The results have been nothing short of eye-opening. They have revealed significant performance gaps for the FunQA videos, exposing weaknesses in spatial-temporal reasoning, visual-centered reasoning, and even free-text generation. This highlights the unique challenges posed by these surprising videos and the pressing need to enhance the depth of video reasoning.
FunQA holds tremendous potential for advancing the field of video understanding. As researchers strive to bridge the gap between artificial intelligence and human-level comprehension, this benchmark becomes an invaluable resource. It inspires and incentivizes the development of novel techniques and models that can unlock the secrets behind our enjoyment of these captivating videos.
MagicQA:Completing the trio of captivating subsets in FunQA is MagicQA. This subset comprises 1,669 mesmerizing videos that showcase a variety of mind-boggling magic tricks and illusions. Magic, with its ability to deceive our senses and defy our understanding of the natural world, presents a unique challenge for video reasoning. It requires the model to not only identify and analyze the visual elements of the tricks but also unravel the underlying mechanisms behind them.
MagicQA videos range in duration, providing a diverse set of challenges for models to tackle. From quick sleight-of-hand tricks to elaborate illusions spanning several minutes, these videos demand spatial-temporal reasoning capabilities from the models. Understanding the sequence of events, identifying hidden elements, and grasping the logic behind the tricks are crucial aspects of unraveling the mysteries presented by these videos.
The inclusion of MagicQA in FunQA enriches the dataset by introducing a new dimension of video understanding. By testing a model's ability to reason through counter-intuitive magical effects, it offers an opportunity to enhance the depth of video comprehension and expand the boundaries of what AI can achieve.
Novel VideoQA Tasks
To thoroughly evaluate the video reasoning abilities of models, FunQA introduces novel VideoQA tasks specifically tailored to this dataset. These tasks go beyond simple comprehension and encompass various aspects of video understanding.
One such task involves counter-intuitive timestamp localization. Models are challenged to identify and locate specific moments in the videos that defy common sense or contain unexpected elements. This task tests the model's ability to pinpoint the exact timestamps where counter-intuitive events occur, requiring precise temporal reasoning.
Another task focuses on providing detailed video descriptions. Models must generate comprehensive and accurate descriptions that capture the essence of the videos, including the key events, actions, and contextual information. This task evaluates the model's capacity to extract relevant information from the videos and construct coherent textual representations.
Furthermore, FunQA presents tasks that revolve around reasoning about counter-intuitiveness. Models are tasked with identifying and explaining the counter-intuitive aspects of the videos, showcasing their ability to detect incongruities between expectations and reality.
Additionally, higher-level tasks challenge models to attribute fitting and vivid titles to the videos, demonstrating their understanding of the content and their creativity in summarizing it concisely. Models are also evaluated on their ability to score video creativity, showcasing their capacity to recognize and appreciate innovative and imaginative video content.
By encompassing a wide range of tasks, FunQA encourages the development of models that excel not only in specific areas of video understanding but also in holistic reasoning and creative interpretation.
the FunQA dataset, with its three captivating subsets—HumorQA, CreativeQA, and MagicQA—paves the way for advancements in video reasoning. By emphasizing counter-intuitiveness, spatial-temporal reasoning, and visual-centered comprehension, FunQA challenges existing models and inspires the development of innovative techniques. With its extensive collection of videos and diverse QA tasks, FunQA unlocks new dimensions of video understanding and creativity, bridging the gap between artificial intelligence and human-level comprehension
Read More:
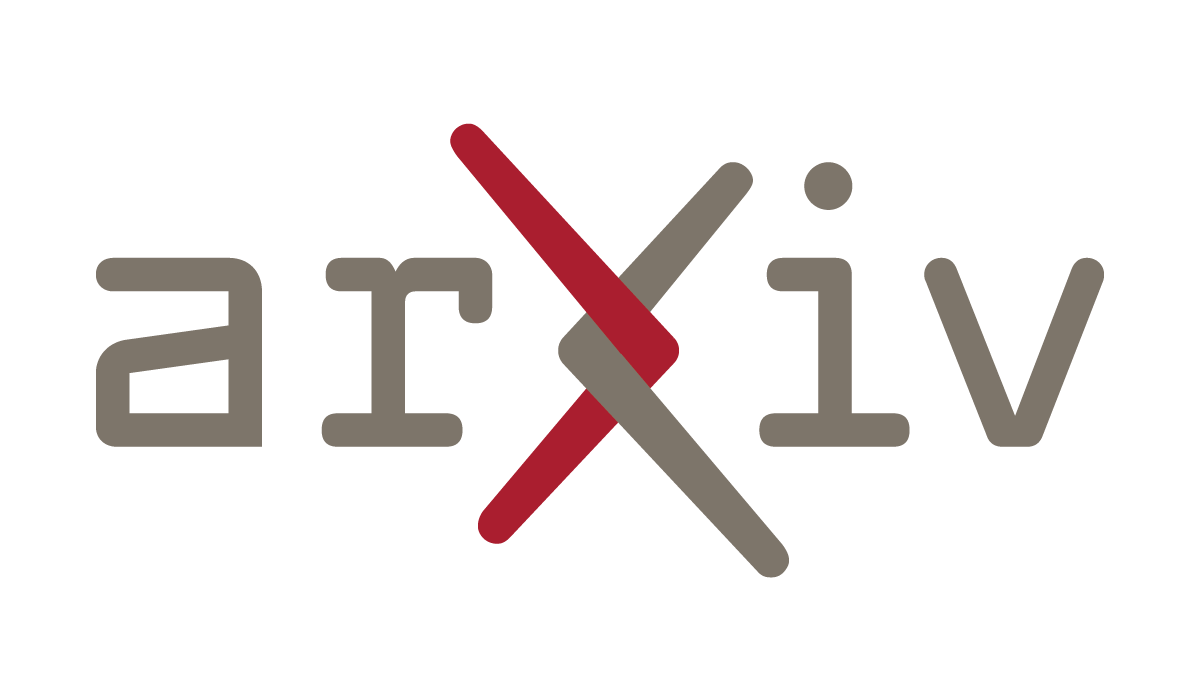
We research, curate and publish daily updates from the field of AI. Paid subscription gives you access to paid articles, a platform to build your own generative AI tools, invitations to closed events, and open-source tools.
Consider becoming a paying subscriber to get the latest!
No spam, no sharing to third party. Only you and me.