Responsible AI
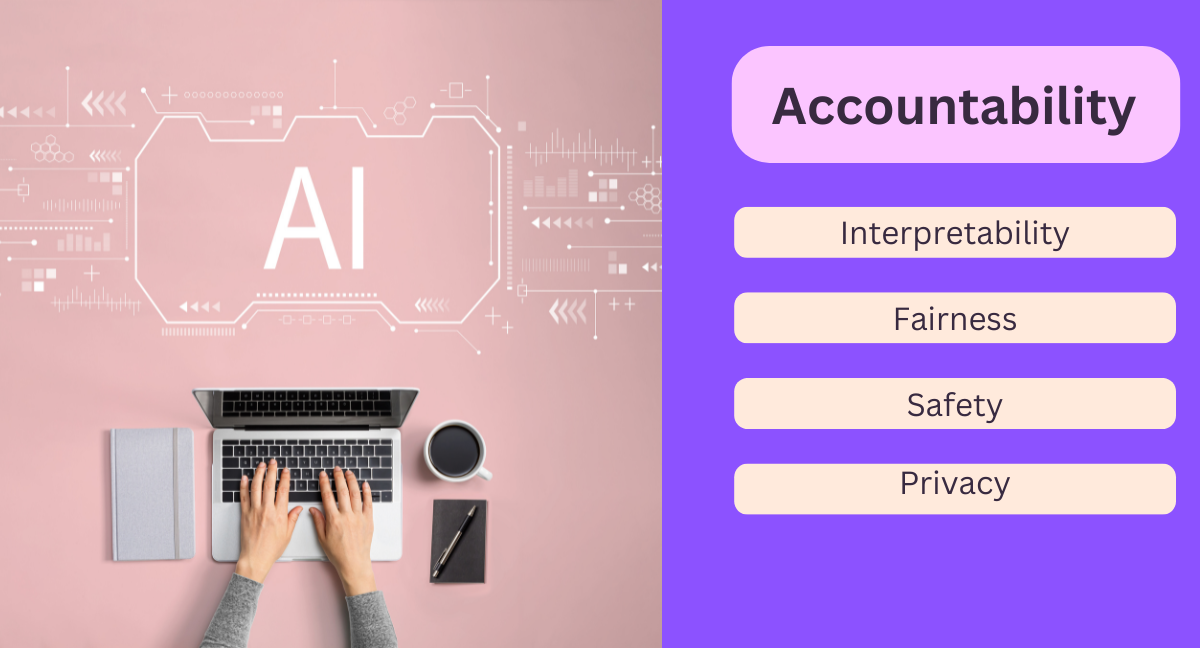
The world is witnessing an extraordinary boom in artificial intelligence (AI) and super-intelligent computation. This AI revolution is reshaping technology, information exchange, and connectivity, setting the stage for a transformative future. Among the various branches of AI, generative AI stands out as a powerful force that is redefining success and progress across all sectors, from medicine to manufacturing. By leveraging deep learning models, generative AI enables the generation of text, images, and other media based on raw data and prompts. These systems possess the remarkable ability to continuously learn and adapt as they receive more data, opening up endless possibilities. However, the rapid development and deployment of generative AI also come with immense responsibility.
Unleashing Creativity and Productivity:
Kevin Scott, Chief Technology Officer for Microsoft, envisions generative AI as a catalyst for unleashing humanity's creativity. He believes that this technology will unlock new ways of iterating faster and create opportunities for productivity enhancement. The potential applications span various domains, including video editing, scriptwriting, drug molecule design, and 3D model-based manufacturing recipes. Both Microsoft and Google have made significant strides in AI technology, integrating generative AI into their platforms and paving the way for innovation.
The Need for Responsible AI:
While the promise of generative AI seems boundless, it is crucial to address concerns about fairness, equity, and safety in its development. One key concern is the creation of AI systems that deliver equitable and unbiased results. An illustrative example is Amazon's attempt to automate recruitment using AI. The company developed an AI system to identify top talent from resumes based on historical hiring data. However, due to the inherent biases in the historical data, the system began favoring male candidates, reflecting the industry's historical gender disparity. Recognizing the implications, Amazon discontinued the program to ensure fairness and transparency.
The Role of Data in AI Development:
The incident involving Amazon's AI recruitment system underscores a fundamental truth about AI: its efficacy depends on the quality and fairness of the data it learns from. Biased or skewed data can perpetuate existing inequalities and hinder progress. In response to this challenge, Google has taken a proactive approach. During its annual developer conference, the company emphasized "responsible AI" as a critical priority, reassuring the audience of its commitment to addressing the potential pitfalls of AI development.
Embracing Responsible AI:
Responsible AI requires a multifaceted approach that involves various stakeholders. Developers must scrutinize training data for biases and work towards inclusivity and diversity. Collaboration with ethicists, sociologists, and domain experts can help identify potential risks and guide the development process. Transparency and accountability should be inherent in AI systems, ensuring that their decision-making processes are understandable and justifiable. Moreover, continuous monitoring and auditing of AI systems are vital to detect and mitigate biases and unintended consequences.
Regulatory Frameworks and Collaboration:
- Addressing Concerns: The rise of AI has brought forth a range of concerns, including unfair decisions, job displacement, and issues surrounding privacy and security. These concerns are unique to AI and require specific attention. Responsible AI aims to tackle these concerns head-on and establish accountability for AI systems.
- Interpretable Models and Fairness: Interpretability plays a vital role in Responsible AI. Users demand explanations for decisions made by AI systems, even if those decisions are correct. The framework can define how to build interpretable models and specify when it is acceptable to use less interpretable ones. Model fairness is closely related to interpretability, as AI systems can exhibit biases stemming from biased training data. Responsible AI frameworks need to address how to evaluate fairness and rectify any bias, particularly when using less interpretable models.
- Safety and Security: Safety and security are concerns inherent in software development, but AI systems introduce unique challenges. Unlike traditional computer systems, AI systems are non-deterministic and can make unexpected decisions or be manipulated to make incorrect ones. This aspect becomes critical when dealing with applications such as self-driving cars, where errors can lead to serious consequences. Responsible AI frameworks should address safety and security concerns specific to AI systems.
- Privacy and Data Governance: Data quality is paramount for AI systems, as mistakes in the data can lead to erroneous decisions. Responsible AI guidelines generally discourage the use of sensitive data and emphasize the importance of data governance. Regulations like the General Data Protection Regulation (GDPR) in Europe provide guidelines for data protection, but companies outside of Europe may need to establish their own data governance practices within their Responsible AI frameworks.
- Building Trust: Trust is a crucial factor in the acceptance and adoption of AI systems. Users will not trust AI systems that use the information they are uncomfortable sharing or that they perceive as biased. Building trust involves providing explanations for decisions and establishing accountability. Responsible AI frameworks can contribute to building trust by ensuring transparency, fairness, and ethical considerations in AI systems.
- Self-Regulation and Regulation: Currently, companies are expected to self-regulate their AI practices by implementing their own Responsible AI guidelines. However, there is a need for consistency across industries and enforcement of these guidelines. The adoption of common Responsible AI guidelines, such as the European Commission's Ethics guidelines for trustworthy AI, can help ensure a standardized approach. The movement towards regulation is gaining momentum, with proposals for new regulations in Europe and calls from tech company executives for increased regulation in the US.
- Algorithm Fairness: Algorithm fairness is a crucial aspect of Responsible AI and is likely to be a focal point in AI regulation. Bias in machine learning models and decision-making processes needs to be understood and mitigated. Algorithm fairness aims to prevent discriminatory outcomes and ensure equitable AI systems.
To fully realize the potential of generative AI while safeguarding against its risks, the establishment of regulatory frameworks becomes imperative. Governments, industry leaders, and academia should collaborate to create guidelines that promote responsible AI development. These frameworks should prioritize transparency, fairness, and ethical considerations, offering a balanced approach that fosters innovation while safeguarding societal well-being.
We research, curate and publish daily updates from the field of AI. Paid subscription gives you access to paid articles, a platform to build your own generative AI tools, invitations to closed events and open-source tools.
Consider becoming a paying subscriber to get the latest!
No spam, no sharing to third party. Only you and me.