Are We Cracking the Code of AI Interpretability?
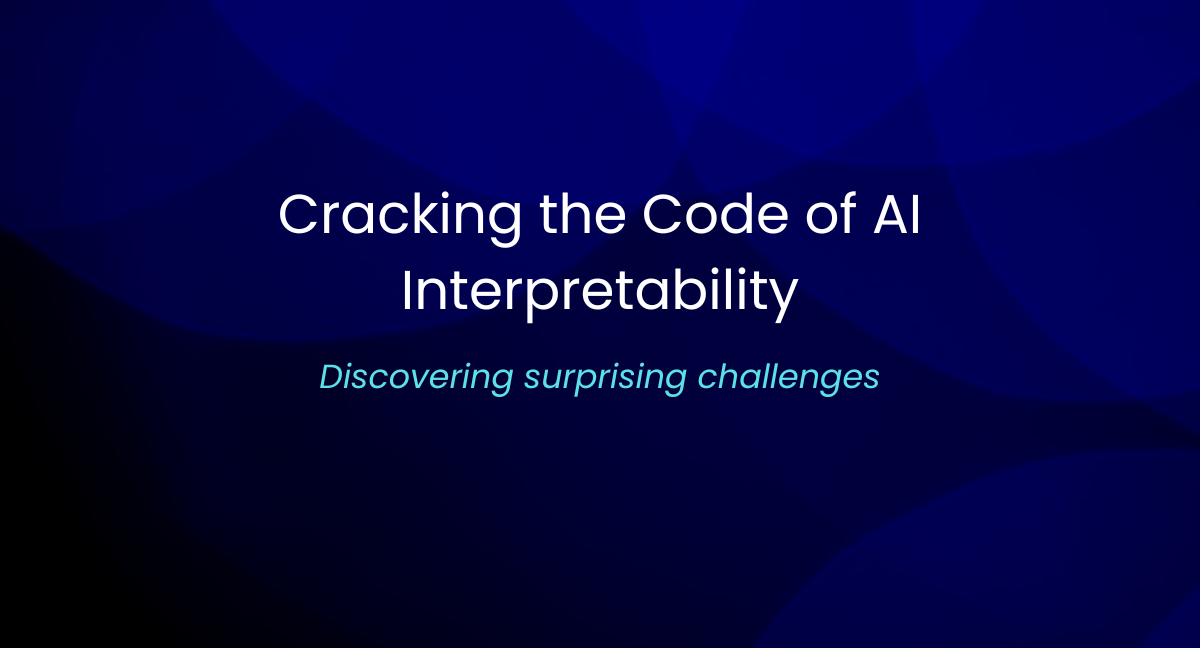
Discovering Surprising Challenges
When it comes to artificial intelligence (AI), the quest for interpretability is a hot topic. Understanding how AI models arrive at their decisions is not only crucial for trust and accountability but also for ensuring that they can be effectively used in various domains. In a recent study, MIT researchers have explored the intricacies of AI interpretability, revealing that it might not be as straightforward as initially thought.
The Complexity of AI Interpretability
In the quest for AI interpretability, researchers have often resorted to techniques designed to make AI model decision-making processes more transparent. However, the MIT study uncovers the intricacies and challenges that lie within this endeavor.
Here are key takeaways from the study:
- Intricate AI Models: Contemporary AI models, particularly deep neural networks, are incredibly intricate. With millions of parameters and intricate layers, unraveling precisely how these models arrive at decisions is no simple feat.
- Balancing Act: Striving for greater interpretability often comes with trade-offs. Simplifying AI models to enhance interpretability can lead to a reduction in overall performance, limiting their real-world utility.
- The Interplay of Interpretability and Performance: Achieving the right equilibrium between interpretability and performance is a delicate dance. Models that prioritize interpretability may not achieve the same level of performance as their less interpretable counterparts.
Real-World Ramifications
The findings of the MIT study carry substantial implications for the AI community:
- Trust and Accountability: While interpretability is undeniably vital for trust and accountability, it may not always be attainable without sacrificing performance. Striking the perfect balance becomes paramount.
- Ethical Considerations: In domains where transparency and fairness are non-negotiable, such as healthcare and finance, navigating the trade-offs between interpretability and performance is a complex ethical challenge.
- Continued Exploration: Researchers must persevere in their quest to uncover innovative methods and techniques that enhance AI interpretability without compromising the effectiveness of these models.
Charting the Path Forward
As AI continues to shape our world, the pursuit of interpretability remains a central challenge. The MIT study underscores that achieving true AI interpretability is more intricate than initially imagined. It's a dynamic arena of research where finding the elusive equilibrium between transparency and performance is the key to unlocking the full potential of interpretable AI.
Understanding the complexities of AI interpretability is vital for the future of AI applications. While it may not be as straightforward as hoped, continued research and innovation hold the key to unlocking the full potential of interpretable AI.
Read More:
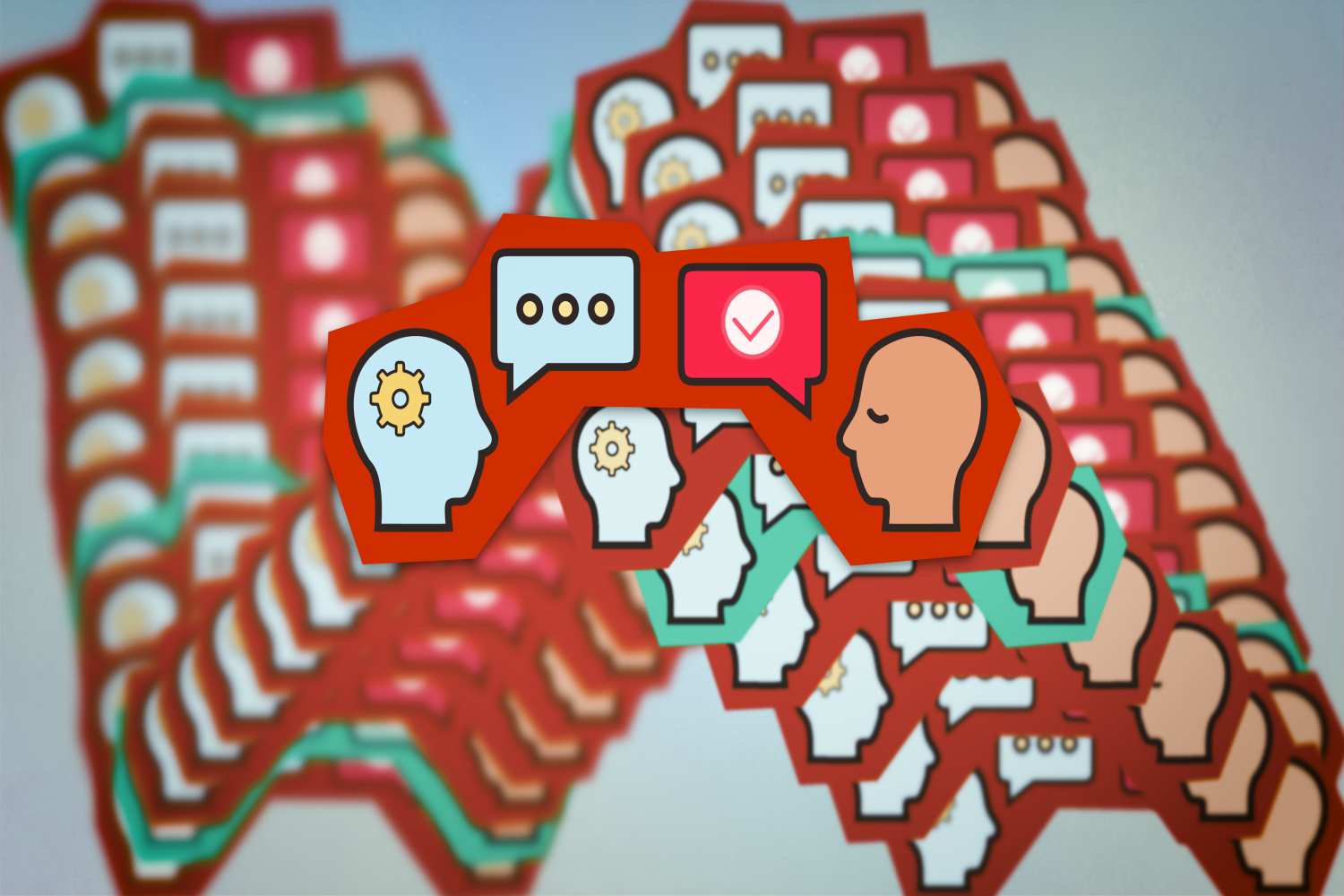
We research, curate, and publish daily updates from the field of AI. A paid subscription gives you access to paid articles, a platform to build your own generative AI tools, invitations to closed events, and open-source tools.
Consider becoming a paying subscriber to get the latest!
No spam, no sharing to third party. Only you and me.