AudioPaLM, a groundbreaking large language model, has emerged as a game-changer in the field of speech understanding and generation. By seamlessly integrating text-based and speech-based language models, namely PaLM-2 and AudioLM, AudioPaLM presents a unified multimodal architecture capable of processing and generating both text and speech. This cutting-edge model offers a range of applications, including speech recognition and speech-to-speech translation.
One of the key strengths of AudioPaLM lies in its ability to preserve paralinguistic information, such as speaker identity and intonation, inherited from AudioLM. Additionally, it harnesses the linguistic knowledge found exclusively in text-based large language models like PaLM-2. By leveraging the best of both worlds, AudioPaLM achieves remarkable results in speech-processing tasks.
A significant breakthrough is observed when AudioPaLM is initialized with the weights of a text-only large language model. This approach effectively capitalizes on the extensive amount of text training data utilized during pretraining, bolstering its performance in speech-related tasks.
As a result, the model surpasses existing systems in speech translation tasks and even demonstrates the ability to perform zero-shot speech-to-text translation for numerous languages, including those not encountered during training.
Furthermore, AudioPaLM exhibits notable characteristics of audio language models, such as transferring a voice across languages based on a briefly spoken prompt. This capability opens up new possibilities for seamless and natural cross-language communication.
In the realm of speech-to-speech translation, AudioPaLM shines brightly. It excels in preserving the original speaker's voice even in the translated audio, providing an unparalleled level of authenticity.
By maintaining the speaker's voice throughout the translation process, AudioPaLM enhances the overall user experience and fosters smoother cross-cultural communication.
To demonstrate the power of AudioPaLM, experiments were conducted using the CVSS-T dataset. Language groups were carefully selected, and representative utterances were chosen for subjective evaluation. The results were astounding, showcasing the model's proficiency in preserving voice characteristics and producing high-quality translated audio.
While AudioPaLM's remarkable performance in speech-related tasks is noteworthy, its impact extends beyond translation. With its impressive advancements in speech understanding and generation, AudioPaLM holds tremendous potential in various fields, including virtual assistants, transcription services, and audio content creation.
As technology continues to evolve, models like AudioPaLM pave the way for more sophisticated and seamless human-machine interactions. By bridging the gap between text and speech, this innovative language model propels us into a future where communication barriers are dismantled, and global connectivity is fostered.
To learn more about AudioPaLM and its groundbreaking capabilities, refer to this research paper:
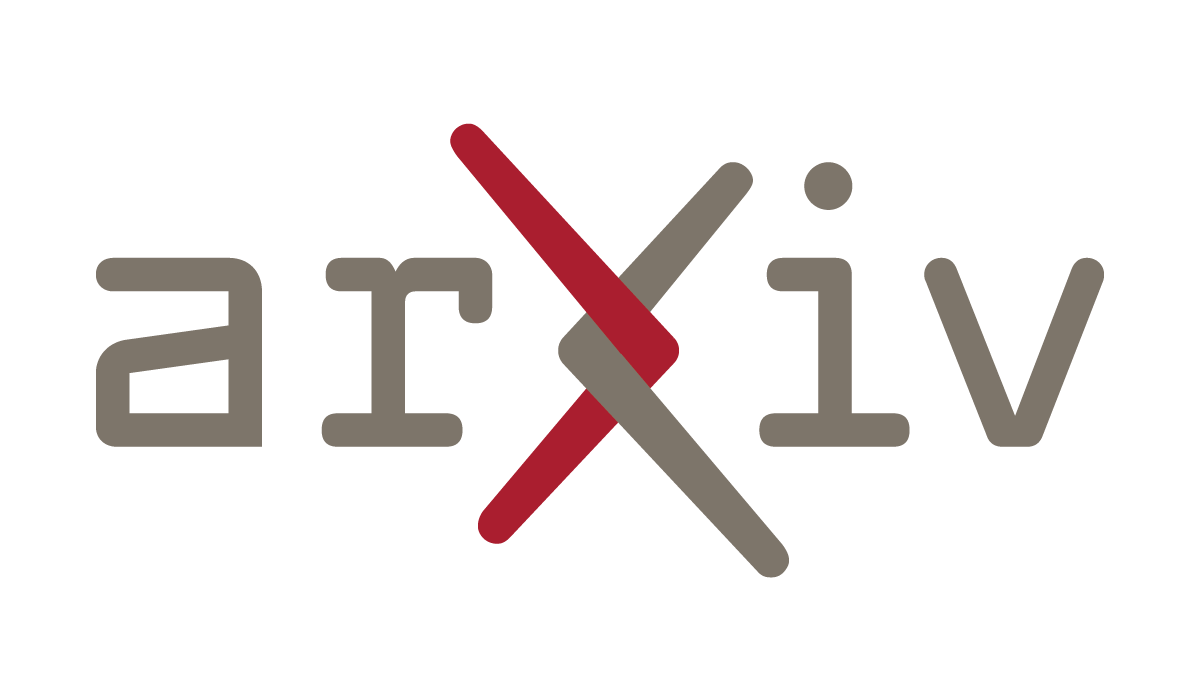
#AudioPaLM #SpeechUnderstanding #SpeechGeneration #AI #LanguageModels #SpeechRecognition #SpeechTranslation #Innovation
We research, curate and publish daily updates from the field of AI. Paid subscription gives you access to paid articles, a platform to build your own generative AI tools, invitations to closed events and open-source tools.
Consider becoming a paying subscriber to get the latest!